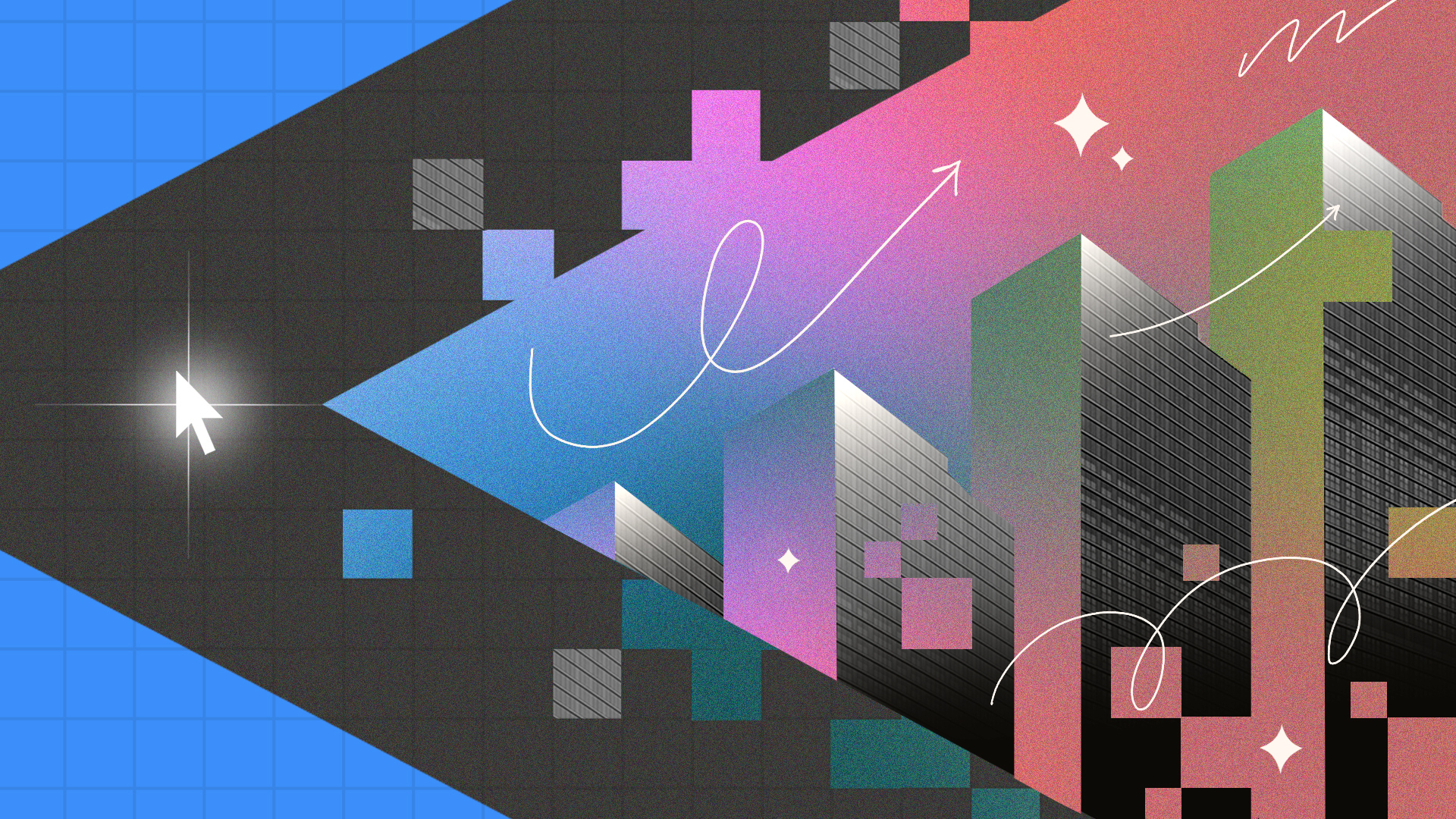
Enterprise AI / Foundational Model Survey
Introduction
Generative AI has quickly moved beyond a consumer phenomenon to making an even larger impact in the enterprise. Companies are now moving to use foundation models across their businesses in a variety of key functions.
We regularly speak with enterprise leaders and recently ran a comprehensive survey with 50+ large companies (>1,000 FTEs) to better understand how they are choosing, budgeting and using the models. The urgency amongst enterprise decision makers is palatable and we have rarely seen large organizations move as swiftly as they have with the adoption of Generative AI use cases. Rather than starting with 1 or 2 use cases, most companies we’ve spoken to have decided to quickly experiment with a broad range of use cases spanning support chat bots, content generation, enterprise search, and contract review among others. That said, many of these use cases currently are still internally focused as enterprise leaders are still looking to better understand the security & privacy challenges and potential quality shortcomings of the current models.
From our conversations it is also clear that the enabling infrastructure for these use cases is still rapidly evolving. While OpenAI had a clear early lead, Anthropic and Google Gemini have since caught up and the open-source ecosystem led by Meta’s Llama and Mistral is following suit. Decision makers are focused on maintaining flexibility by experimenting with multiple model vendors.
To help founders understand how enterprises are deploying AI models and what their needs are, we’re sharing key learnings from the survey as well as from our direct conversations with enterprise AI leaders.
At Lightspeed, we have made significant investments across the many parts of the emerging genAI stack. You can find an overview of some of our investments in this article.
Going Broad—Quickly—Across Many Use Cases
Enterprises – especially large ones – can be notoriously slow at adopting new technologies. Even ~20 years post the launch of AWS, close to ~50% of enterprise workloads have yet to move to the cloud (src: Gartner). The initial adoption of Generative AI use cases has happened at an incredible pace, often driven by top-down mandates from senior company leadership.
The majority of companies in the survey have three or more use cases for foundational models. Rather than slowly introducing new use cases one-by-one, over 60% of respondents in our survey stated that their organizations already have 3+ use cases leveraging Generative AI in some capacity.
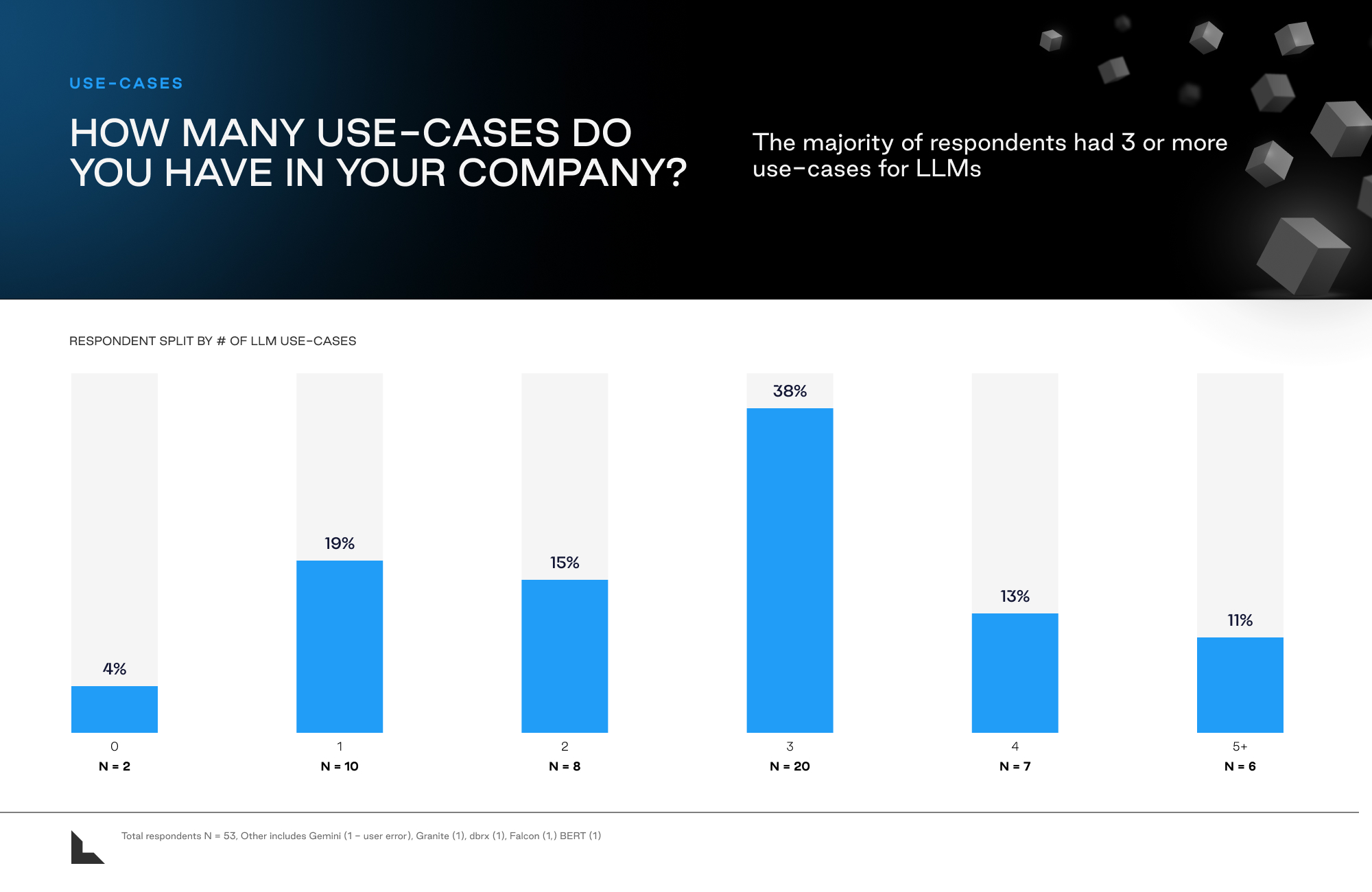
The use cases span everything from text summarization, to internal chatbots, external support chatbots, or enterprise search. Companies are also surprisingly quick at moving use cases into production as decision makers are seeking to understand the potential impact of these solutions quickly.
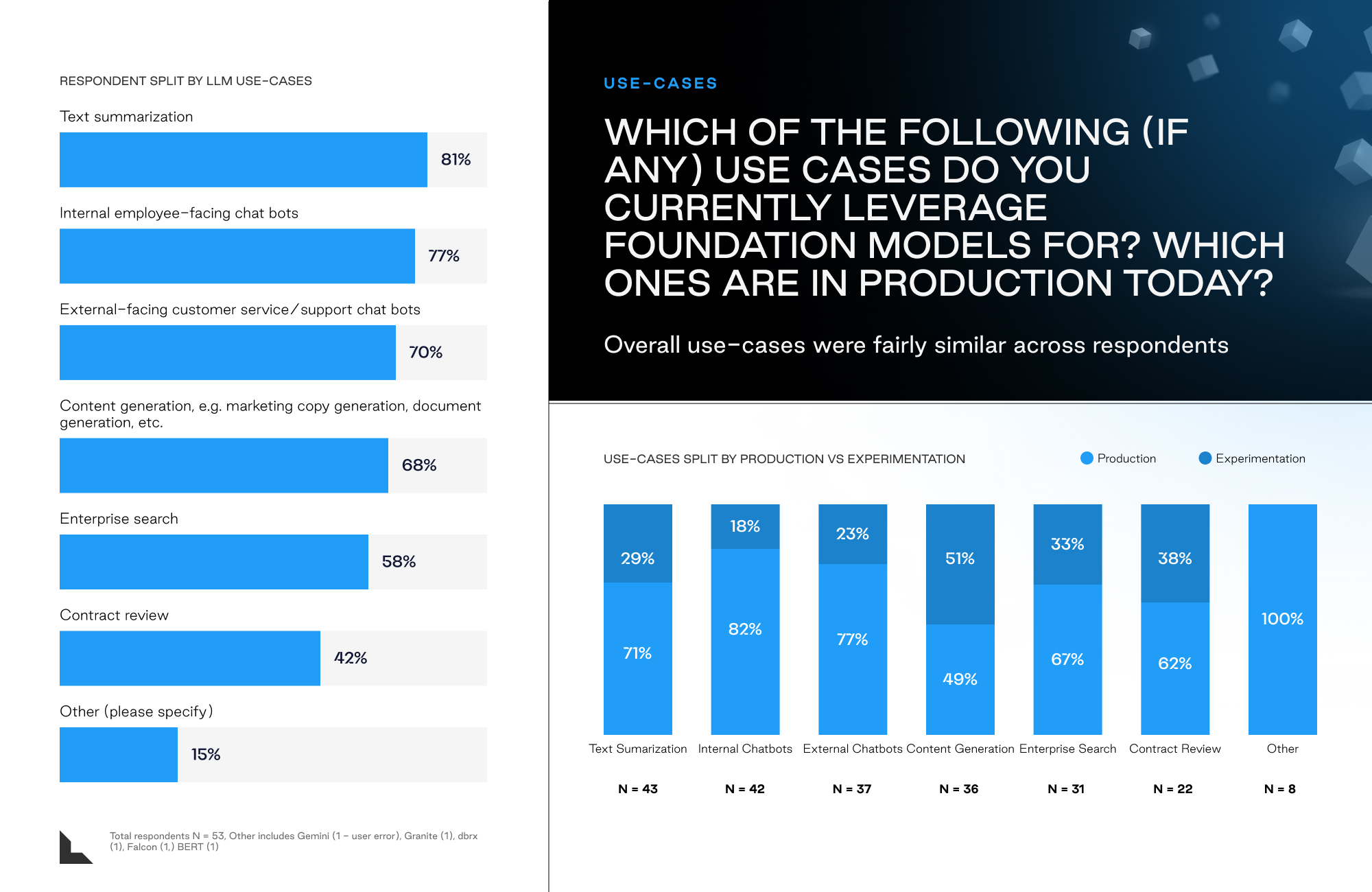
Data Privacy and Performance Stand Out as Key Barriers to Enterprise Adoption
That said, moving this quickly doesn’t come without challenges. The most prevalent concerns we heard in our direct conversations with key decision makers centered around data privacy and underlying performance:
LLMs open up new potential data privacy pitfalls:
“Data privacy remains an issue, how do we make sure our models aren’t biased, providing proprietary information, or wrong answers to our customers, employees and stakeholders” (Large Public Market SaaS Platform)”
“Our challenges in scaling LLM usage is not so much from the tech perspective, but more from the data privacy and security perspective. We have lots of data privacy and requirements across our different business jurisdictions on what can / cannot be shared and figuring out how to ensure compliance with our LLM applications is still unclear” (public workforce productivity SaaS)
LLM applications have yet to consistently cross minimum performance thresholds:
“There’s been significant model improvements across all dimensions (e.g. in latency), but the only thing we’re still focused on is evaluating and hitting acceptable performance criteria…we’re not quite there yet” (financial institution)
“Our criteria hasn’t changed in the past year…quality and performance is still by far the most tracked metrics, everything else are factors, but secondary” (private consumer internet company)
These challenges also clearly came through in our survey:
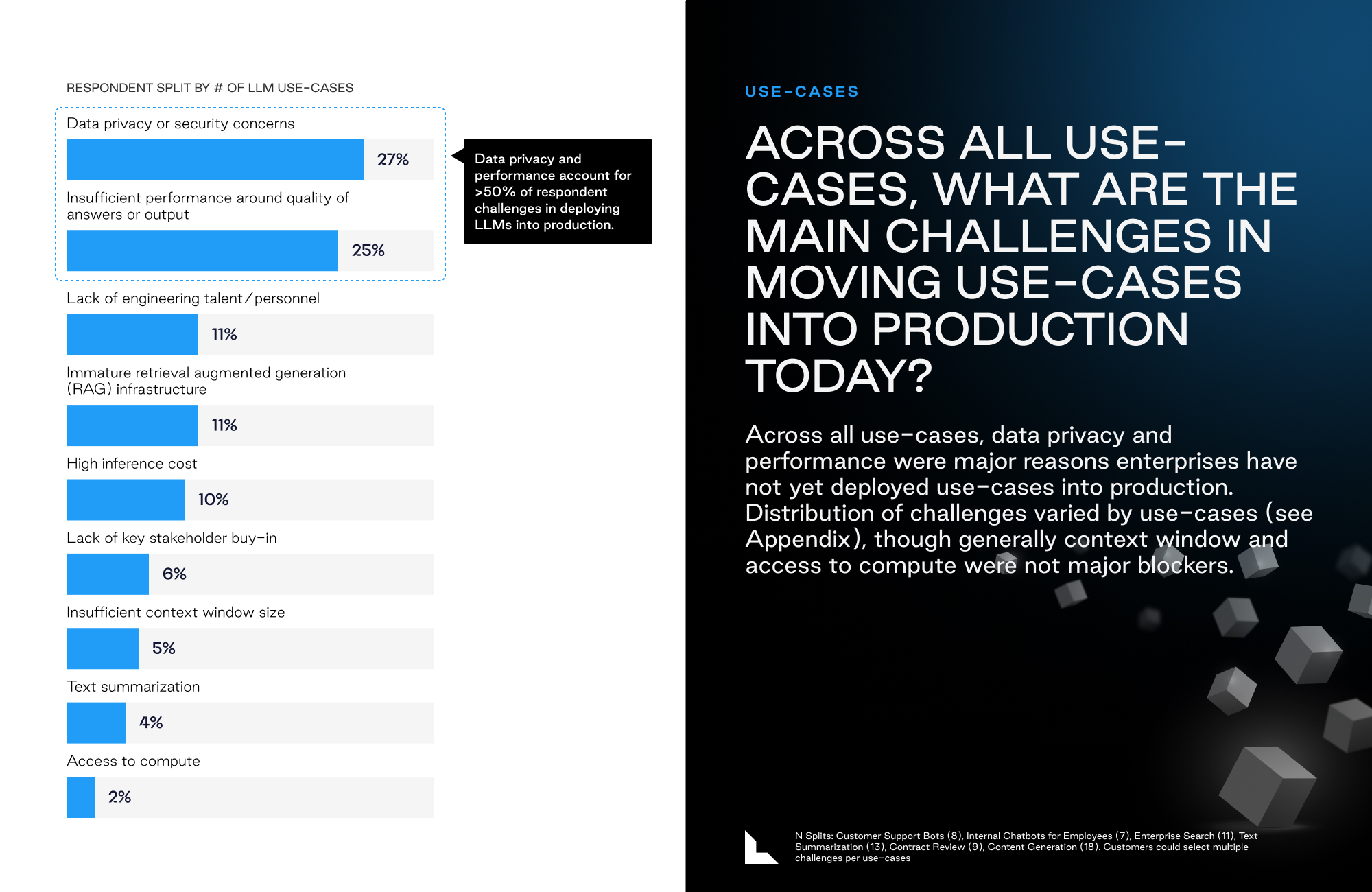
Internal Use Cases Take a Headstart in Response to Production Challenges
As a result of the above concerns, enterprises are focusing on internal use cases first, as they continue to address production challenges and the underlying LLM technology improves. Our survey responses indicated the most common LLM applications were for internal-facing use cases such as text summarization, enterprise search or content generation. One notable exception was the high enterprise interest in developing chatbots (both internal and external), though this may be due to enterprises perceiving LLMs as an augmenting technology on existing customer chatbot systems, vs a net-new use case. However, even within chatbots, internal employee-facing chatbots saw a greater overall presence, suggesting similar concerns around production still hold.
Qualitative commentary from decision-makers validates this dynamic, with enterprises such as a large legal tech company citing the overall immaturity of their LLM and Generative AI landscape as a reason behind their more cautious approach towards external, customer-facing use cases:
“We have a number of internal use case we’ve been working on, but not really any external ones that touch customers…we have questions around data privacy and we want the landscape to mature before we bring this to customers” (legal tech company)
These challenges to enterprise LLM adoption are also being recognized across adjacent industries. For example, at the most recent 2024 RSA conference, a survey found that LLM and AI security was the #1 top priority amongst C-suite decision-makers, with a number of new startups emerging to address LLM data privacy issues in response to market demand.
Rapidly Changing Enabling Infrastructure Layer
When ChatGPT was first released in November 2022, Open AI’s GPT-3 and subsequent GPT-3.5, GPT-4 and Turbo series of models had established themselves as the clearly dominant LLM models / vendor. While other vendors released various competing models in 2023, there was a notable gap relative to OpenAI’s latest offerings. However in 2024, that has changed dramatically, with the recent launches of Anthropic’s Claude 3 and Meta’s Llama-3 significantly disrupting the existing LLM landscape. While OpenAI today is still the most widely used model, Anthropic has caught up quickly with its Claude 3 family of models, and numerous enterprises surveyed are now expecting to adopt Anthropic and/or shift usage away from OpenAI in favor of Anthropic. Additionally, open-source has been receiving a lot of attention with the recent Llama-3 announcements and Mark Zuckerberg’s public commitment to open source, and most importantly, a willingness to invest significant amounts of capital behind it. As a result, Llama-3 is now the most popular OS model followed closely by Mistral.
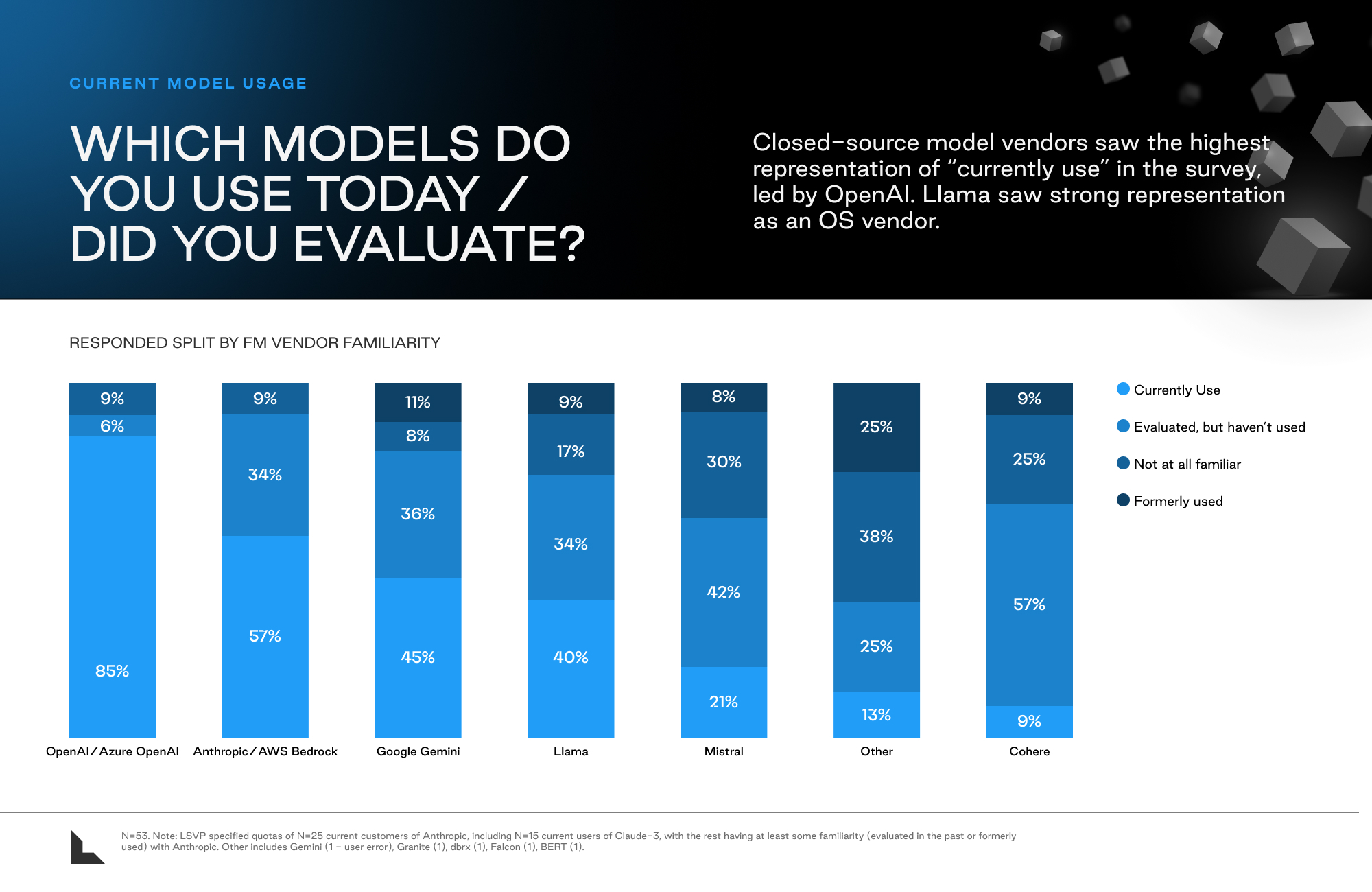
OpenAI Has First-Mover Advantage in Production, but Others Are Challenging
Our survey work showed that OpenAI continues to be the dominant vendor for production use cases, though this is probably at least partially a function of the 1+ year head start that OpenAI’s GPT-4 and GPT-4 Turbo had over newly comparable models such as Claude 3. In our conversations with practitioners over the past few weeks we have noticed a clear shift and the recognition that there are now viable alternatives to OpenAI’s models.
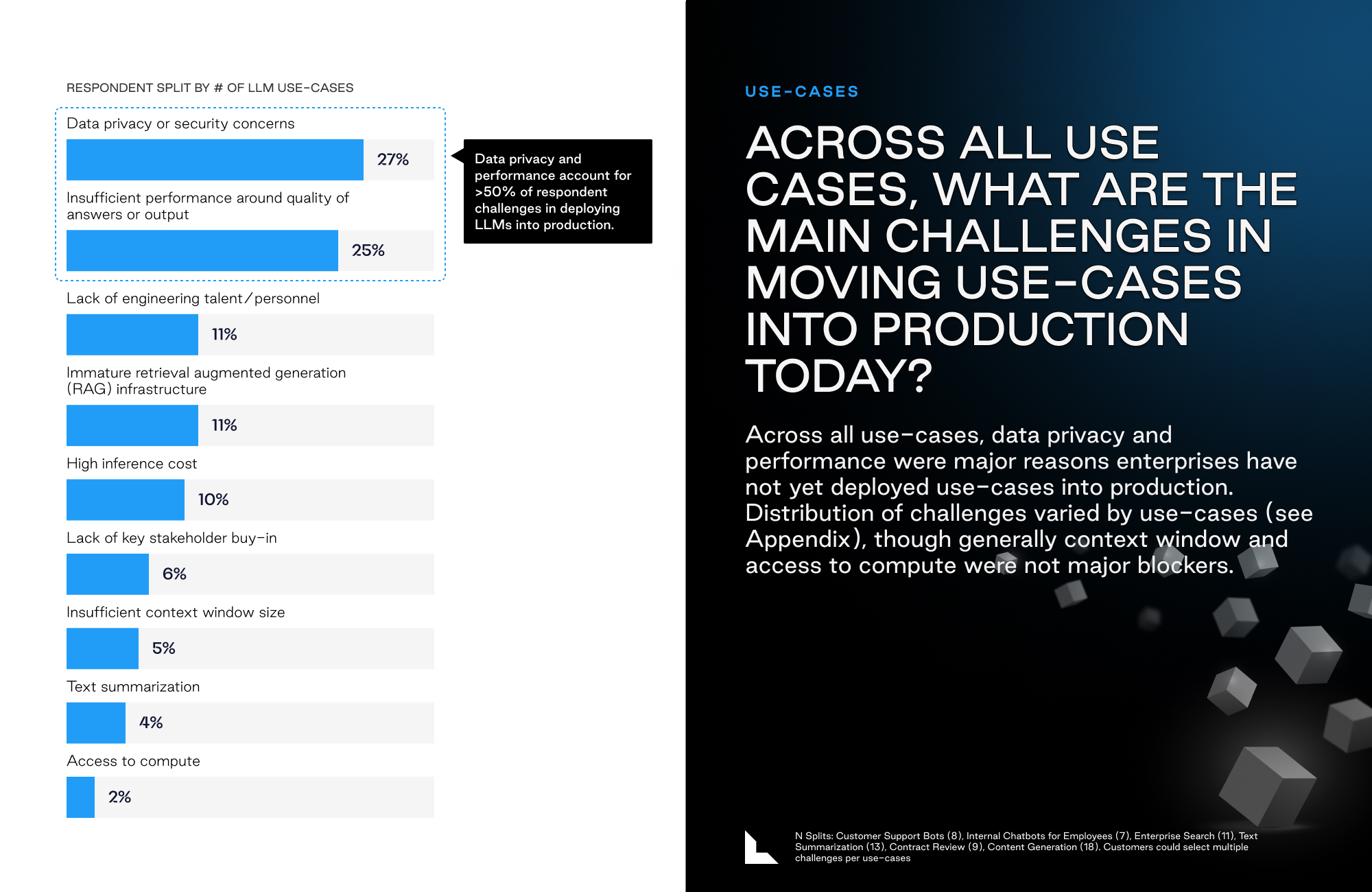
Respondent feedback on Anthropic’s latest Claude 3 models has been very positive, with most enterprise decision-makers viewing Claude 3 as a major step-change improvement over Claude 2. More importantly, most experts also view Claude 3 as comparable to GPT-4, which crowns Anthropic as the first model vendor to “close the gap” with OpenAI and offer a comparable model.
“Claude 3 is a huge change relative to Claude 2, it has improved on every dimension…Anthropic is now faster than GPT-4 and at least as good if not better on quality depending on use case” (financial services)
“Personally, I feel like Claude-3 has really closed the gap with GPT-4 on quality, so you’re just choosing based on other secondary criteria” (workforce productivity SaaS)
“We’re being careful not to commit too deeply to any model vendor…but my gut reaction is that Claude 3 will now shift more [spend] to Anthropic over OpenAI” (workforce productivity SaaS)
We expect that the foundational model space will continue to be extremely dynamic. A small group of vendors has emerged as having the internal capabilities to build leading edge models and for the medium-term future we’d expect to see the various models take the lead for a period of time. For enterprises, the name of the game will be flexibility.
Enterprises Are Responding by Staying Flexible
Today, there is not yet an established set of infrastructure components or vendors in Gen AI, and the space is rapidly evolving. From the technology side, companies do not want to rely on just one foundation model or model vendor in case new developments in the space render their existing investments obsolete. As discussed above, the past few months have overseen significant upheaval in the LLM vendor landscape with the releases of Claude 3 and Llama-3, and we expect the dynamic market conditions to continue into the foreseeable future. Even just the anticipated releases of GPT-5 (or GPT-4o as it turns out) and the Llama-3 400B model are expected to cause another reshuffling in model rankings, much less any additional models or technical breakthroughs that may be further out on the horizon.
On the use case side, most enterprises are also still in the discovery phase of determining the highest ROI use cases for LLMs within their existing businesses and the exact form-factor those applications should take. Even for companies that have established an early framework for LLM applications, decision-makers will still want to multi-source model vendors both to optimize model choices to their respective use cases, and also to protect against any unexpected model failures or downtime.
Given these two considerations of a dynamic market landscape and overall nascency of use cases, most enterprises recognize the value in staying flexible and keeping their options open. Our survey results indicate that very few (<5%) of respondents single-source to one model vendor, and the average company has 2.3 model vendors today in production, with potential to increase in the future.
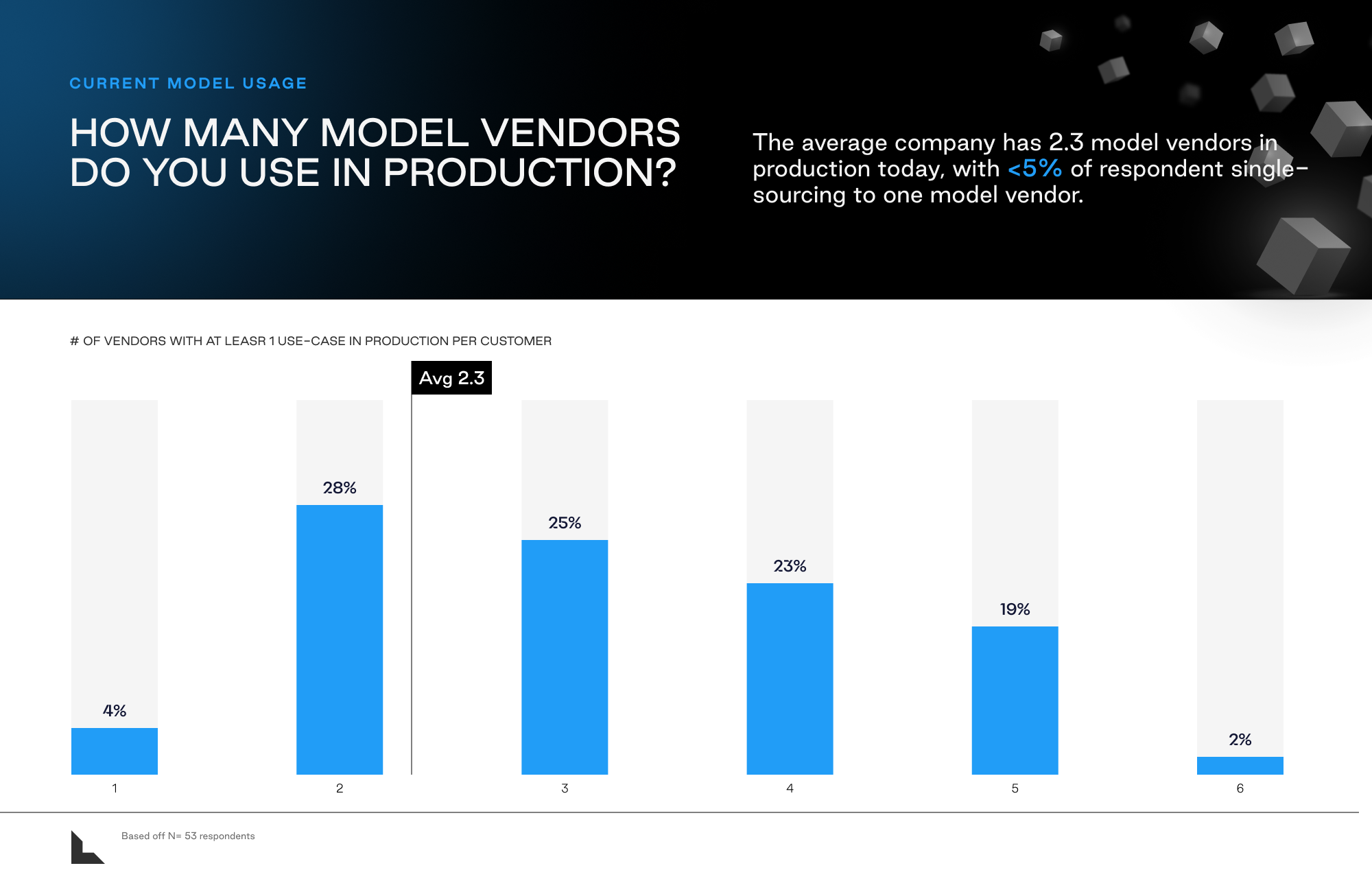
New Models Are Adopted Quickly in a Dynamic Market
One interesting dynamic we have observed is that both the release and the uptake of newest models are extremely fast, perhaps in contrast to other traditional infrastructure segments. Lightspeed’s view is that at this period in the market’s evolution, because the newest models have significant step-function performance improvements over their predecessors, it’s often still worthwhile for companies to churn off older model versions in favor of the latest model. For example, despite the Claude 3 family of models only being launched recently in March 2024, its adoption has already surpassed that of the Claude 2 generation (43% vs 31%, respectively), which was launched almost a year ago in July 2023.
The rapid adoption of the latest model versions may also partially be explained by the overall nascency of enterprise production use cases, where companies can more easily upgrade to the latest model version when applications are still being tweaked in experimentation.
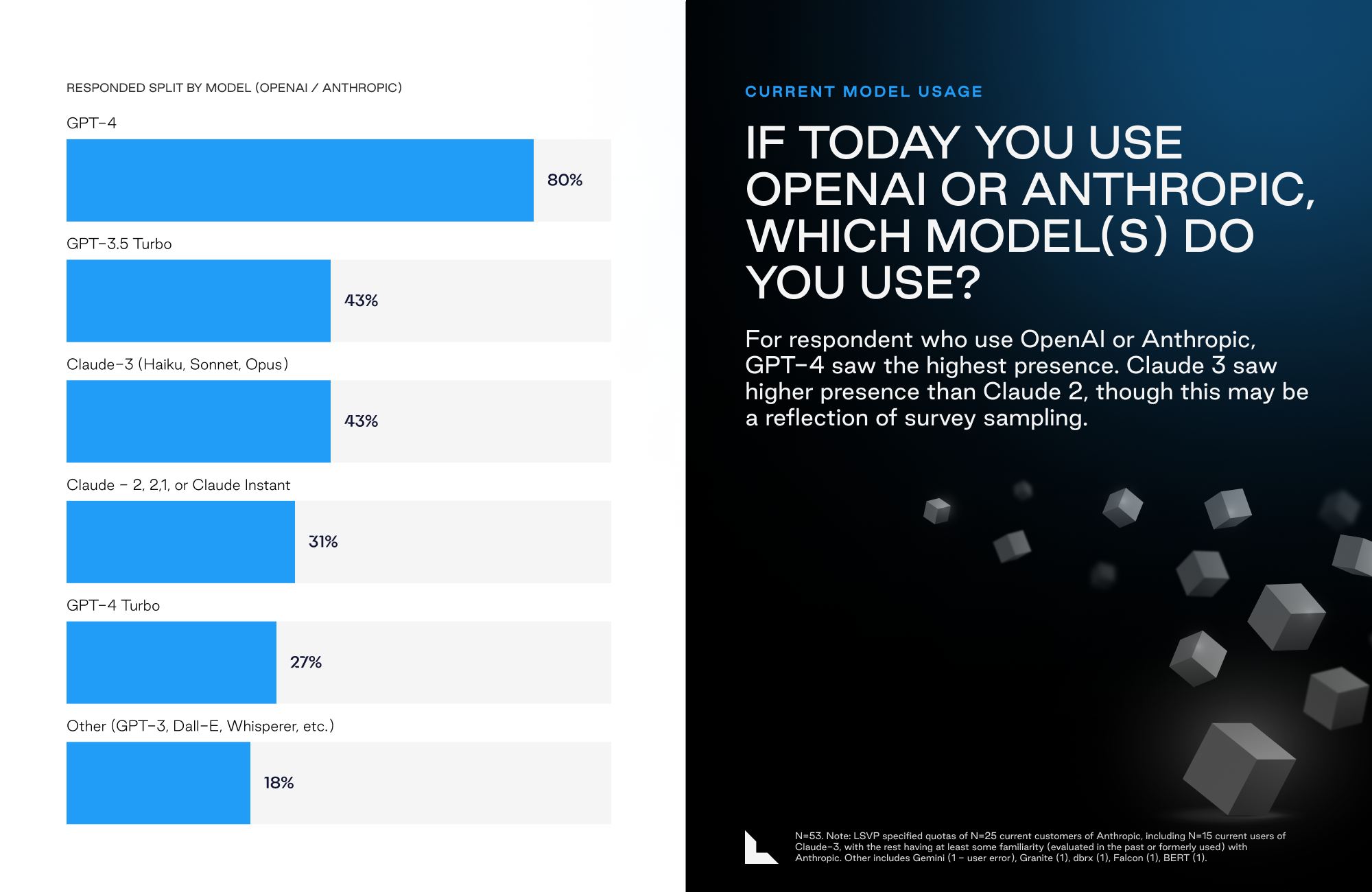
Hyperscalers as Model Kingmakers?
The medium and long-term evolution of the LLM market is difficult to predict, given the rapid developments in the space. However, one view of the world is that the foundational model space will play out similarly to the cloud infrastructure world and the hyperscalers end-up as “Kingmakers” in the category.
In the early days of LLMs, companies seeking to leverage models would generally interface directly with the model vendors themselves, either through an API call or in some instances, a custom fine-tuned model. Today, the market has evolved to support three primary types of consumption models: direct from model provider, through a third party inference specialist, or through a cloud provider.
The direct from model provider approach is largely similar to the early days, where a customer directly consumes a vendor like OpenAI or Anthropic through their API. Some vendors have begun offering specialized access or tiered pricing (e.g. removing rate limits, dedicated inference instances).
A number of specialized third party inference vendors such as Modal and Fireworks have similarly emerged, primarily to simplify customer adoption of open-source LLMs. In the same way that OpenAI provides API access to their models, these third party specialists provide API access to popular open source models such as Llama-3. These vendors also offer fine-tuning services for customers to customize open-source models as well. Enterprises leveraged specialized inference providers primarily to optimize for speed in building out experimental use cases, whereas individual developers were drawn to the sleek and easy-to-use developer experience.
On the cloud provider side, we have seen the hyperscalers move aggressively to partner with model vendors or in some cases, develop their own LLMs (Microsoft with OpenAI, Amazon with Anthropic, Google with Gemini) so that the models can be consumed through a customer’s existing cloud environment. By consuming LLMs through their existing cloud provider, customers can leverage their existing infrastructure setup (e.g. storage and data ingestion) to more easily deploy LLMs. Additionally, customers are also able to benefit from the existing data security / privacy and general enterprise controls (e.g. identity and access) within their cloud environment. Lastly, cloud providers are also enabling customers to pay for LLM usage with their existing cloud compute credits, which greatly simplifies the customer billing and procurement process. All these benefits combined suggests that we should expect to see a significant number of enterprise customers consume LLMs through their existing cloud provider both today and over time, which we see validated in our data across all vendors.
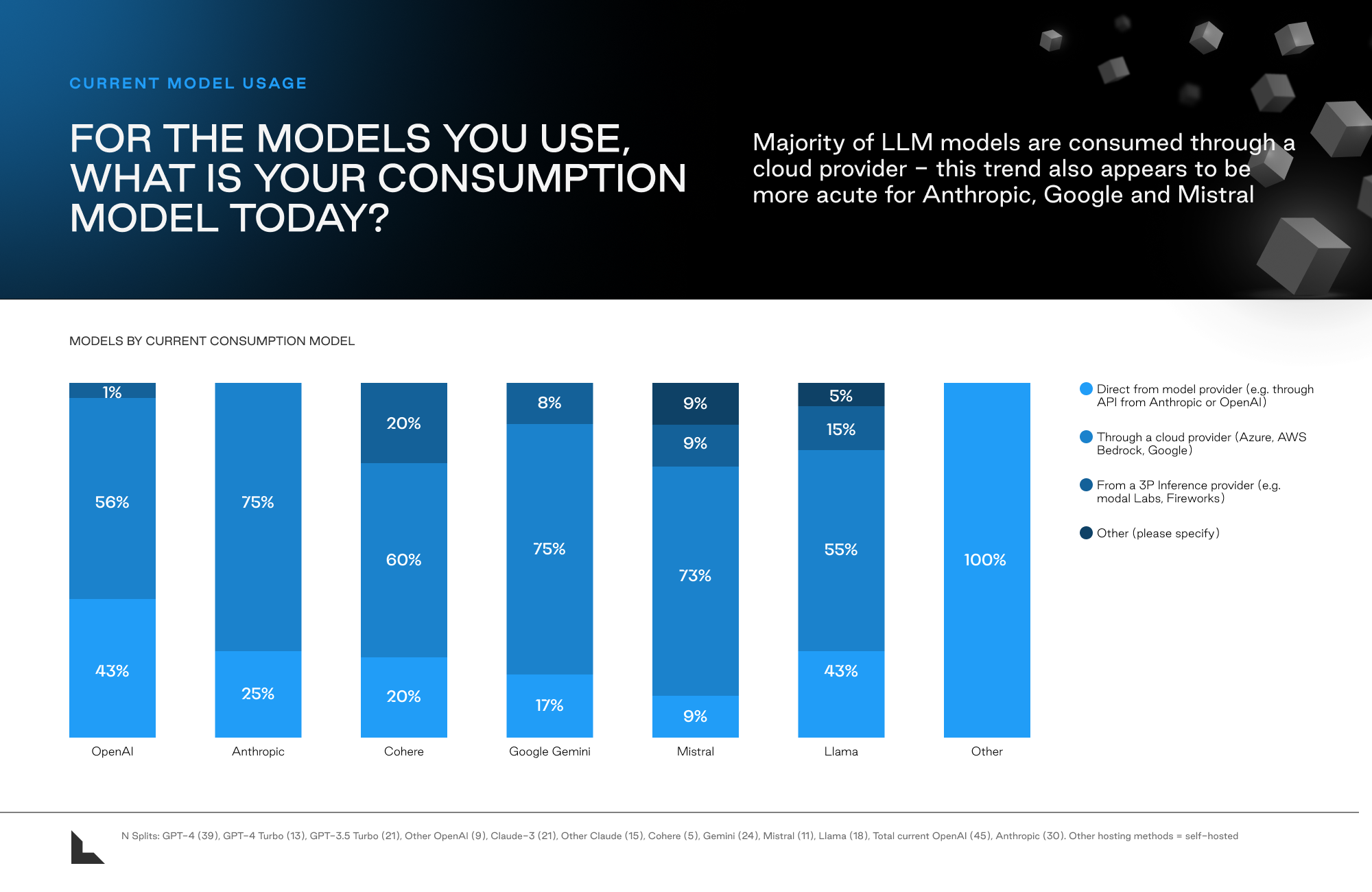
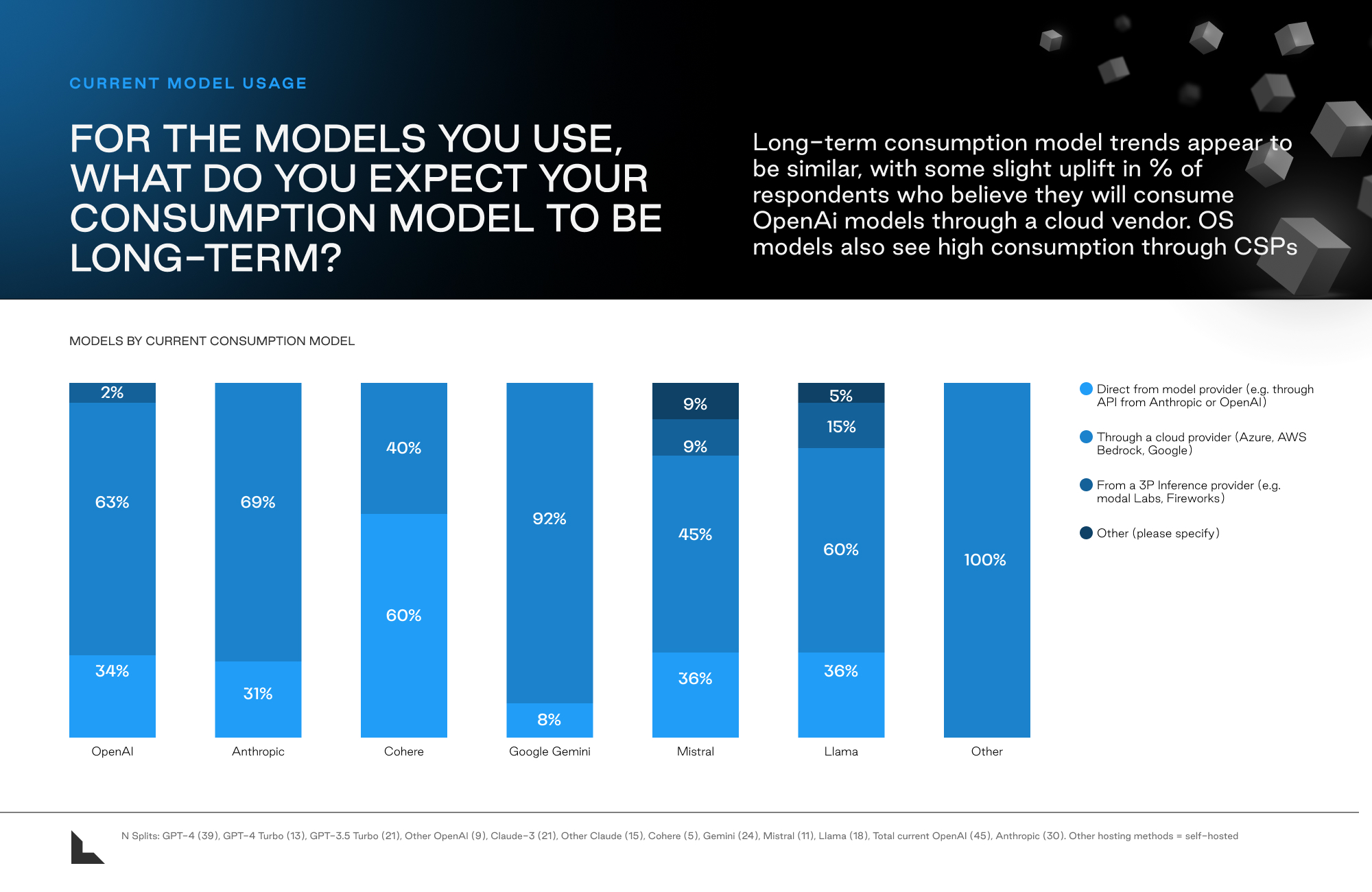
Given the strong and growing popularity of consuming LLMs through a customer’s respective cloud provider, we believe it’s possible that the evolution of the LLM market will align with their respective hyperscaler partner. In other words, an enterprise using Microsoft Azure will be more likely to use OpenAI and an enterprise using Amazon AWS is more likely to use Anthropic as long as there is no significant performance gap between models available through the different hyperscalers.
Some Friction in Switching Between Models
While enterprises are clearly still maintaining their flexibility when it comes to model selection, there are some initial signs that some stickiness could emerge once the pace of change in model performance starts to slow down. In our discussions with executives, they’ve told us that while switching can be done, there are several reasons that doing so presents challenges.
First, prompts are designed and optimized for specific models and aren’t completely transferable. And after switching models, a company will have to re-evaluate and optimize its tests—but the required tooling and frameworks to do so also aren’t always compatible between the different vendors.
Furthermore, it takes time and engineering resources to prepare the supporting infrastructure, such as data ingestion, to import data into LLMs and handle LLM outputs. And they also aren’t necessarily transferable between models.
What’s more, certain companies have specific proprietary data that requires customization to fine-tune the models. That work would all need to be redone when switching to a different model. And certain models have features or advantages over others, such as support for agentic workflows or larger context windows. The foundational model vendors will no doubt try to expand on features that can increase the lock-in into their models.
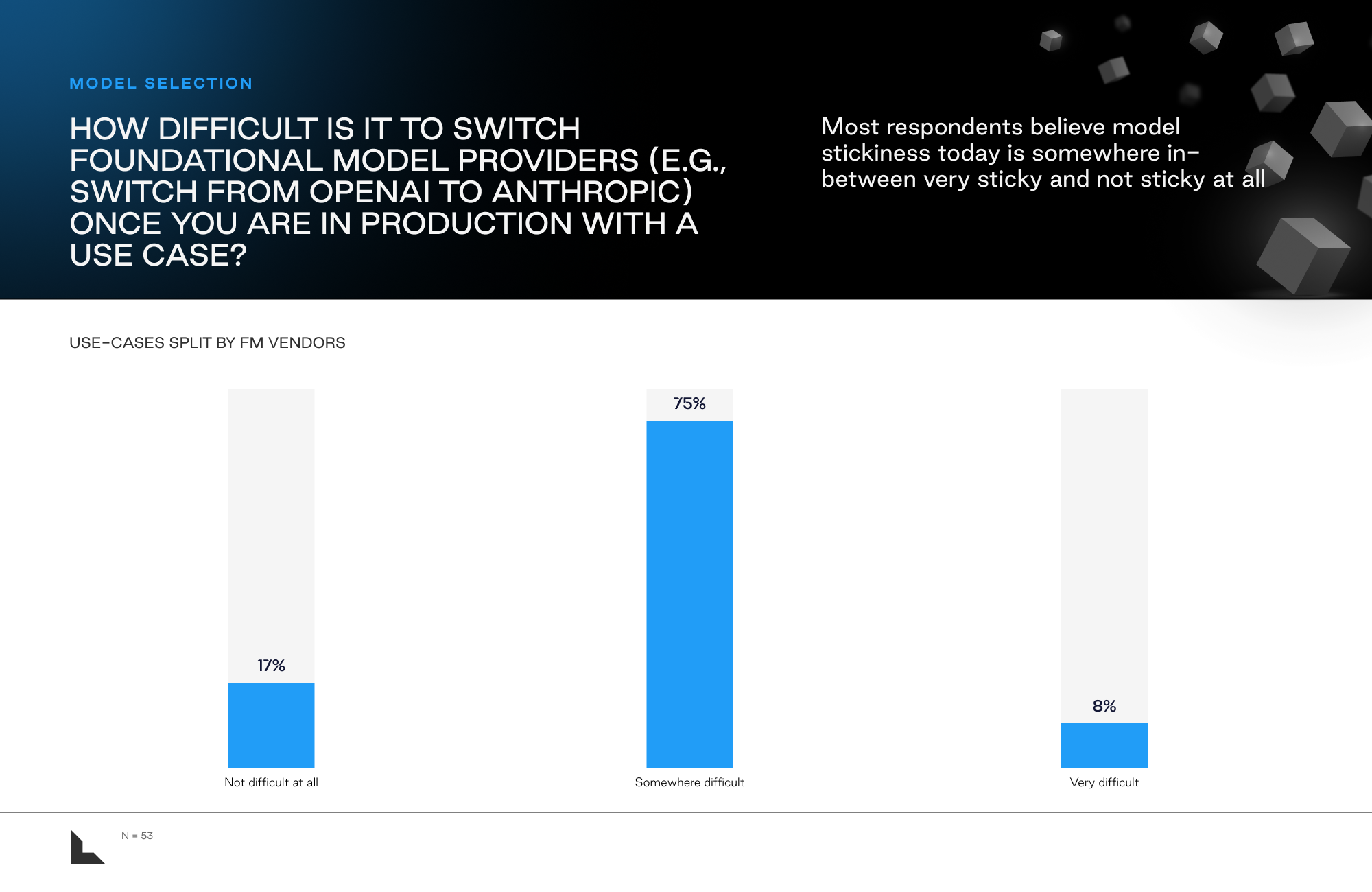
Enterprises Already Dedicating Significant Budgets to Foundational Model
As a measure of how much of a priority enterprises are placing upon Generative AI, companies are already making large financial commitments to models today. About 25% of respondents spend more than $3 million in 2023. That number is expected to rise to nearly 50% in 2024 (as a reminder, our survey targeted companies with >1,000 FTEs). As noted above, foundation models are increasingly moving from experimental to production uses cases and budgets are growing as a result. One cautionary note for vendors outside of GenAI is that the spent on foundational models (and related infrastructure) has at least partially been cannibalizing other budgets.
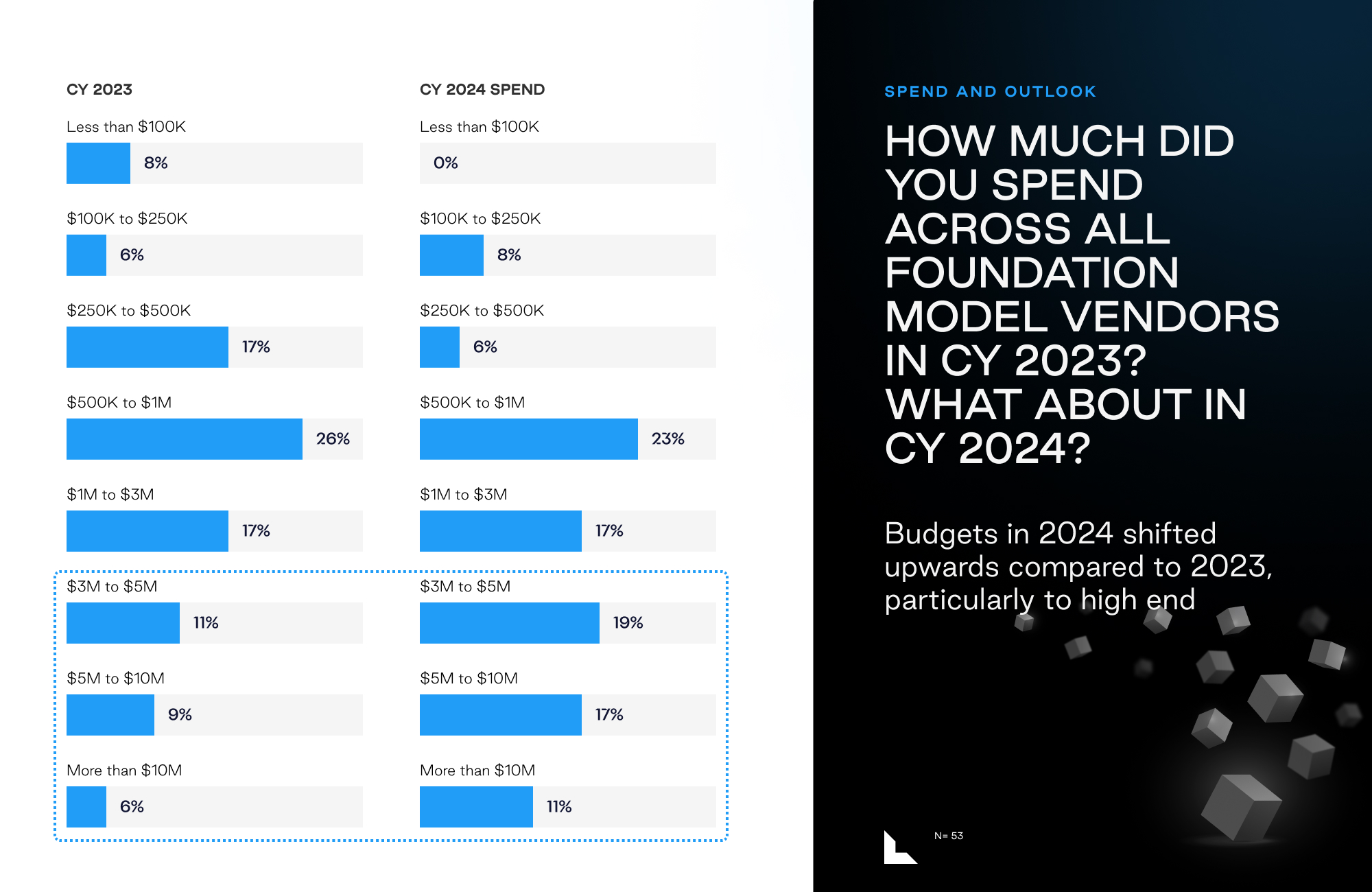
Authors