While today’s leading financial services businesses undoubtedly benefit from operating in one of the largest addressable markets, they also must contend with some of the most stringent and costly regulations in modern history. Companies serving the financial needs of enterprises and consumers alike are facing an increasingly punitive environment – global penalties for failure to comply with AML, KYC, ESG, and related regulations totaled $6.6B in 2023, up considerably from $4.2B in 2022 and $5.4B in 2021. Notable recent enforcement actions include a $1.3B U.S. Department of the Treasury fine against TD Bank for insufficient AML procedures and a Federal Reserve warning to Evolve Bank to bolster its risk management programs around fintech partnerships and AML.
With bank-fintech partnerships coming under an unprecedented regulatory microscope over the last few years and financial institutions reporting a further $10B in annual revenue lost to inefficient onboarding processes, the imperative for better compliance enablement tools has never been more pressing.
AI is poised to overhaul the status quo in FinServ compliance
While headlines of massive fines and sweeping new regulations clearly underscore the need to leverage technological innovation into more robust and proactive compliance solutions, most institutions still rely on a jumbled mix of manual processes and outdated Governance, Risk, and Compliance (GRC) software. In fact, 28% of organizations still rely on paper-based methods to track compliance data, and though software solutions abound for compliance needs, current tools are largely reactive in nature, complex, expensive, and often ill-suited to the rapidly expanding needs of today’s compliance teams.
We believe the compliance use case is particularly well suited for an AI-enabled overhaul. In contrast to other enterprise problem areas, most compliance tasks are relatively deterministic (e.g., “Does this transaction match our definition of potential money laundering?”, “Have we collected all of the necessary customer data to comply with our KYC requirements?”), with clear yes or no answers. The bulk of today’s compliance costs are driven by manual workflows, such as document review and online investigations– expensive, time-consuming, and labor-intensive processes in desperate need of intelligent automations. Managing the modern compliance workflow has only become harder over time with the exponential growth of disparate data sources, third-party integrations, and relevant regulations.
So far, startups are taking an AI-first approach to automating outdated compliance workflows. The most common focus areas we’re seeing include:
- Enhancing pre-existing workflows for new client onboarding and transaction monitoring (KYC/KYB, AML)
- Bolstering continuous monitoring capabilities (automated horizon scanning, obligation management, third-party marketing review)
- Strengthening employee oversight (trade monitoring, communications review)
Though the latest innovations have coalesced around the same few mission-critical workflows/use cases, the technological approach to addressing them varies:
- AI-enabled SaaS (AI model underlying automated processes)
- Specialized agents and co-pilots (auto-complete, autonomous task execution)
- AI-enabled chatbots (largely Q&A-driven)
Mapping the market: Where we see opportunity
Below, we segment the AI compliance landscape into its primary focus areas and explore where emerging innovation is already making a difference.
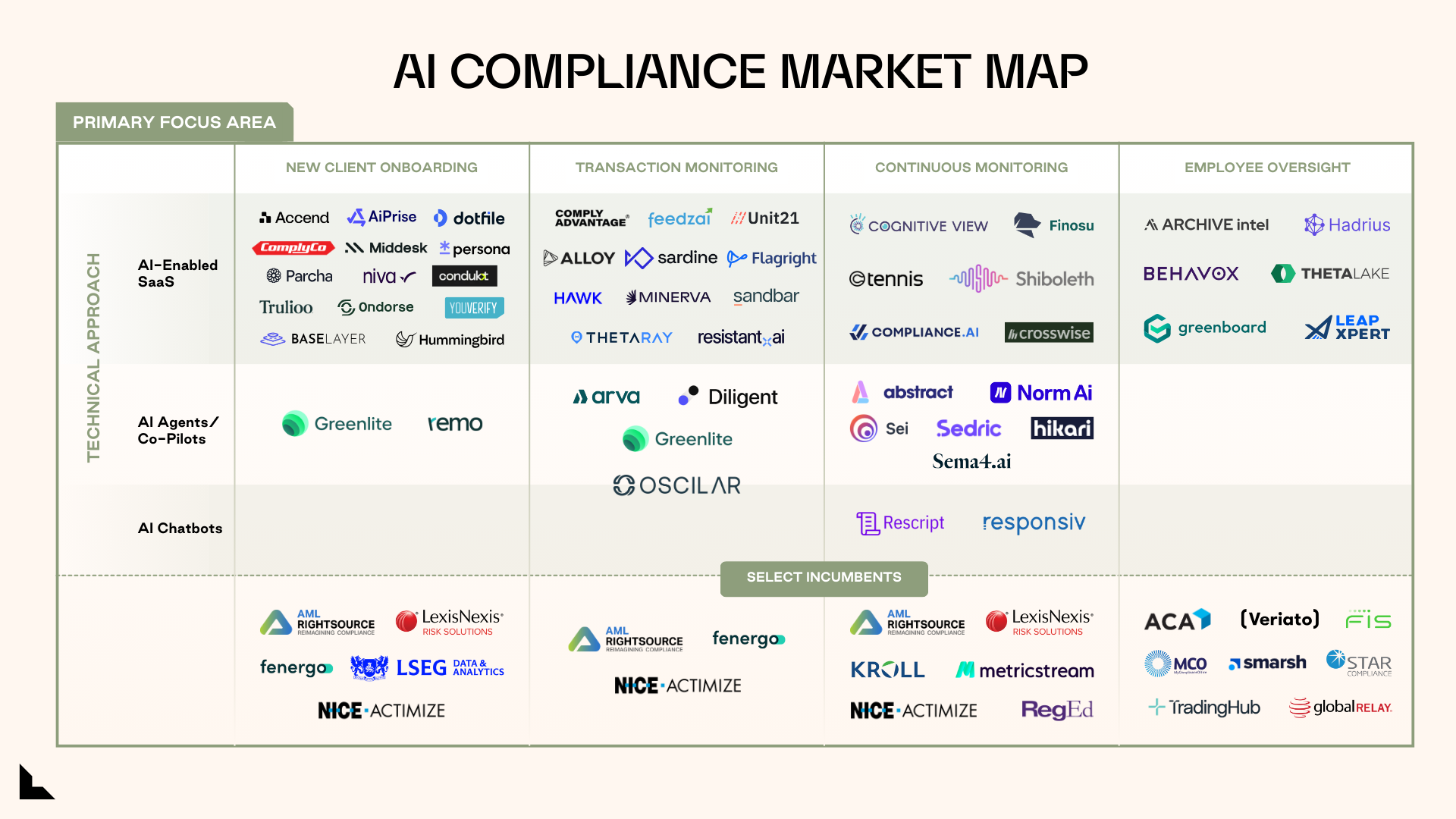
New Client Onboarding
Appropriately onboarding new customers through KYC/KYB verification has historically been one of the most manual and time-consuming tasks within the finserv sector, requiring extensive documentation and cross-checking to meet regulatory standards.
Legacy incumbents such as LexisNexis Risk Solutions search a database of millions of structured individual and business profiles in order to verify identities. These solutions do not extend to the broader web sources (e.g., LinkedIn, private databases) relied upon for verifying recently created businesses and typically require additional human intervention to fully close out compliance checks. Once the responsibility of banks alone, emerging regulation scrutinizing embedded finance partnerships has magnified the importance of enhanced onboarding automation across the financial services spectrum.
Emerging Innovation: Startups building in the space aim to leverage AI to materially decrease time to customer onboarding without added regulatory risk. By automating data collection, verification, and compliance screening and expanding applicable sources consulted, these emerging onboarding solutions improve efficiency, reduce errors, and enable financial institutions to onboard clients faster and more securely.
While incumbents have streamlined the initial KYB data-gathering process, customers still cite high levels of manual review or supplementation required and are turning to startups such as Parcha to further close the gap with enhanced, AI-enabled business verification, automating a material portion of junior analyst work and building a more robust customer profile for required checks (e.g., KYC, KYB, adverse media screening, etc.). Alternatively, companies like Accend initially differentiate through depth of expertise in unique attributes such as industry risk decisioning and then expand to complementary add-on services beyond onboarding, such as document extraction for underwriting workflows. Startups addressing this challenge tout materially higher accuracy rates and lower false positives than the manual status quo.
We view customer identity verification alone as a low-margin, high-volume commodity service unlikely to drive venture-scale returns. That being said, providing a superior onboarding service as an entry point into the enterprise before expanding into other complementary, more complex services could bolster a strong land and expand case.
Transaction Monitoring
AML tools act as some of the most critical points of defense against reputational and financial damage to finserv organizations. Current AML transaction monitoring software like NICE Actimize primarily leverages rules-based logic and risk-based algorithms to flag potentially risky activities for further manual investigation by internal compliance teams and outsourced organizations such as AML RightSource. Balancing the efficiency-driven desire to reduce false positives and increase volumes with the need to maintain robust controls to detect suspicious activity remains a challenge for providers.
Emerging Innovation: In order to overtake established incumbents specializing in AML compliance, startups are focused on decreasing cost without any loss of fidelity as well as enhancing predictive capabilities through generative AI automation.
Greenlite, for example, is taking an agentic platform approach to addressing financial crime and initial onboarding, specifically focusing on the manual and repetitive tasks keeping L1 analysts from tackling the complex compliance workstreams requiring human judgment. Greenlite customers find the product to be particularly adept at sourcing data from all relevant corners of the internet, with mature enterprise customers increasingly treating it as a mission-critical component of their compliance stack (especially on the enhanced due diligence side for high-risk businesses).
Investors will want to see startups in this space transition from data gathering and facilitating regulatory audit trails to risk decisioning that ultimately defines the strength and effectiveness of an enterprise compliance program.
Continuous Monitoring
Regulatory compliance extends far beyond the initial customer sign-up, with specialized software tools needed for keeping track of emerging relevant regulations (i.e., horizon scanning), monitoring third-party sales and marketing compliance, and real-time reporting on organizational risk. As both regulation and sales and marketing channels have risen dramatically, so too has the need for automated, scalable solutions for comprehensive ongoing monitoring. Solutions like LexisNexis focus on broad-based regulatory coverage and static alerts for pre-existing GRC systems, providing reactive tools in response to evolving regulatory changes and forgoing real-time communications alerts altogether.
Emerging Innovation: Nascent startups are building proactive and real-time response capabilities through AI models fine-tuned to the compliance use case and the financial services regulatory landscape. Companies like Norm Ai are transforming complex regulation into decomposable code for use by AI co-pilots, giving compliance teams the newfound ability to instantly ensure compliance with all applicable regulations both old and new. Alternatively, startups such as Sedric focus more on scaling internal guideline compliance to external sales and marketing partners and real-time violation mitigation at the point-of-sale.
Innovators building here face intense competition from legacy incumbents with similar, albeit less technologically advanced capabilities, and typically go to market with some kind of unique wedge (e.g., Sedric typically onboards banking customers with its third-party debt collection agent compliance solution before expanding to ancillary platform services). While advanced genAI capabilities and a strong focus on UI currently differentiate emerging innovators from incumbents, we have doubts about the long-term moat here as legacy players continue to bolster their tech stacks.
Employee Oversight
Employee oversight solutions span regulatory compliance, insider threat prevention, and sensitive information protection. These tools monitor employee activities, such as communications, transactions, and access to confidential data, across an increasingly broad array of channels, making policy or regulatory violation detection more challenging than ever. Despite making progress in proactive flagging capabilities, incumbents specializing in employee monitoring, such as Smarsh and Global Relay, are ultimately record-keeping solutions with little opportunity to move beyond a commodity offering.
Emerging Innovation: While the communications monitoring itself is undifferentiated, what startups are doing with the comms data post-ingestion is where we’re seeing incremental value add.
Customers utilizing startups like Hadrius cite a staggering 99% reduction in false positives, driven largely by context-aware AI that goes beyond incumbents’ keyword-driven flags to streamline what gets passed on for manual intervention. Further, startups innovating in this area are taking more of a platform approach to employee compliance than legacy players, with embedded offerings spanning trade monitoring, attestations and disclosures, and marketing review.
We believe technical differentiation on the false positives side and better UI for additional platform capabilities will be critical for the emergence of a new player of scale here.
Unanswered questions, present challenges, and opportunities in AI compliance
As we attempt to predict the short- and long-term path forward for AI compliance tools, several important questions remain:
- Regulatory Evolution: How quickly will regulators adapt to AI-based compliance solutions? Startups must find the balance between innovation and regulatory compliance, potentially requiring close collaboration and advocacy with regulators. If the EU AI Act provides any indication of a potential global response, AI output testing will be critical, with stringent requirements placed on the data governance policies determining input data for model training as well.
- Pricing Models and Scalability: Will usage-based pricing or per-user models dominate? The answer will shape the scalability and market reach of compliance tools, particularly for small to mid-sized institutions. While usage-based pricing is likely more advantageous for SaaS providers in this space, software costs must fall materially below the salary expense of human intervention to justify a change to the status quo of how compliance is handled today. With the variable costs associated with AI putting pressure on gross margins, profitability at scale remains a question mark.
- Competitive Moats and Differentiation: Winning solutions will likely be those that combine best-in-class predictive capabilities, strong data integration, and user-friendly interfaces. While finserv compliance is a huge addressable market, it is incredibly crowded with incumbents and startups alike, all tackling similar problems with similar approaches and underlying datasets. Technical differentiation and trust through explainability and embedded testing will be critical to displacing long-entrenched incumbent solutions in such a mission-critical space.
Looking ahead
The demand for efficient, accurate, and forward-looking compliance solutions will only intensify as financial services face higher regulatory pressures and operational costs. To win in such a saturated market, startups must prioritize building a compliance-specific data moat that results in unparalleled retrospective and predictive accuracy as well as a differentiated wedge into the customer’s compliance workflow. Incumbents have been slow to respond to AI innovation to date, giving emerging players a head start in bolstering the depth and breadth of their solution set as well as the technical approach by which they execute on it.
For investors, this market offers a unique chance to support technologies that not only help financial institutions meet today’s regulatory demands but also prepare them for future challenges. At Lightspeed, we are committed to backing companies driving this transformation, and we look forward to supporting the growth of an industry that will ultimately lead to a more resilient, transparent, and secure financial ecosystem.
The Lightspeed Fintech team is actively spending time working with founders in compliance enablement and supporting the next wave of AI innovation. If you’re building here, let us know: reach out to me, Sam Eisler, or my Fintech colleagues, Justin Overdorff and Aaron Frank. Special shout out to Steph Hay for her collaboration on this article.
Authors