There has lately been a lot of talk about capital cycles that are driven by interest rates. Today I want to talk about a different kind of cycle — the innovation supercycle — which I find to be far more interesting and impactful. Understanding the innovation supercycle is important to understand how an innovation gets distributed and commercialized and which assets are going to accrue value and for how long before the next one comes along.

Before we go any further, it’d be appropriate to pause over a few concepts that were introduced to the world by three great macro-economists — Schumpeter, Ricardo, and Malthus. You don’t have to study the above three seminal books cover to cover unless you really want to unpack their ideas, but in short, a highly dumbed down summary of the ideas relevant to this article are below —
- Schumpeterian forces that create what are ‘extractive’ industries — industries that create the new as they destroy the old, strive to produce more with less, and unlock some net-new resource for the world.
- Next comes the Ricardian forces that take this new resource and distribute it to new markets, make this resource accessible to all. Generally these forces deal in trade, expansion, and competitive dynamics.
- Finally, the Malthusian forces create fear that there won’t be enough for everyone. That as resources expand, so shall the need for it, till there isn’t enough for everyone. And thus the cycle goes back to ‘extractive’ — extract something else, extract more, saturate, restart.
In a way they are the triumvirate of macroeconomic trends that rule the world. One creates a new wave, the other runs that wave through its natural lifecycle, and the third destroys and takes us back to creation. As these 50–100 year macroeconomic cycles are playing out, there is an innovation cycle that operates underneath it, subservient to it. This cycle may be 30–40year cycle of capex and opex dominant forces. What does this mean? Every few decades, a few decades worth of CAPEX — time, people, & money put into research, development and innovation — is able to unlock a new ‘resource’ that then eventually becomes ubiquitous and cheap for the world to use and build new things with which then turns into an OPEX cycle. If all this sounds a bit academic, just give me a few minutes and it’ll all make more sense.
The “mega resource” theory of super-cycles
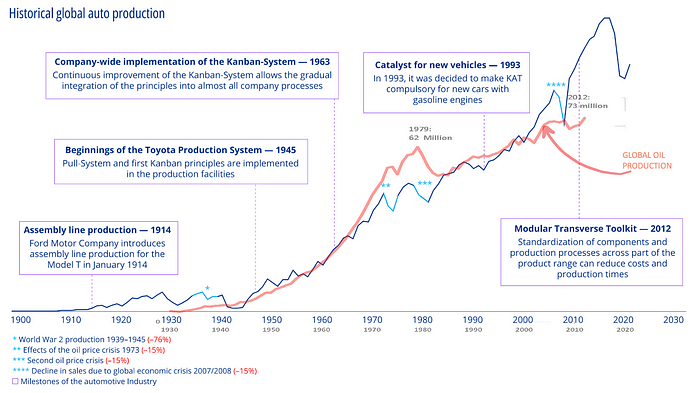
Let’s take a few examples to understand how new resources get unlocked and distributed in the world, starting with the mother of all modern resources: Oil. See above an overlaid graph of global oil (red) and auto (blue) production. During the 1920–1950s, the world was still going through the early phases of the oil-led innovation-CAPEX cycle. Though we had spent 100+ years trying to dig for oil, we were still trying to figure out where and how to dig at scale, scientifically. All this R&D took several decades and by the 1940s, we had gotten so good at it that countries were literally being occupied for it. This was around the time this Schumpeterian ‘extractive’ cycle was somewhat played out and we were entering Oil’s Ricardian OPEX heavy cycle. You can see that in the auto production capacity jumping around 1940s in the graph above, and the distribution efficiency continuing to improve through the next few decades. An OPEX cycle isn’t as much about the de-novo innovation of ‘I have a car and you have just a horse’. It’s more about ‘my car is bigger than yours, I have a nicer chassis, a better brand’ and so on. In other words, not about the innovation as much as a marketing or product spin on that innovation. A 2nd order effect of OPEX cycle in the car industry are things like car chases in Hollywood movies, or James Bond driving my car vs yours, etc.
A similar wave also happened in semiconductor space through the 1960s-90s. Starting with Fairchild, then Intel & AMD, about 20–30 years of R&D went into making a net-new resource – a CPU cycle – available to the world easily and cheaply. As a result, practically every device in the world now has some sort of a “CPU cycle” running under the hood. The initial CAPEX / R&D heavy innovation cycles of the 1960–1990s eventually gave way to more OPEX heavy cycles in the semiconductor industry. When I was at AMD during 2005–2011, the joke was that Intel’s marketing budget was larger than AMD’s entire R&D budget. Sure, we had several ‘mini-R&D/CAPEX’ cycles going on in the semiconductor world e.g. from 32bit to x64 or single to multi-core but broadly the heart of Intel’s message to the market has been “Intel Inside” , not performance or performance/watt which is what you’d focus on if you were to signal true innovation in the category.
And then the 2nd order effect of this net-new resource — the CPU cycle — becoming cheap and ubiquitous was that it kicked off the software cycle. The beginning of the software cycle — let’s just call it the internet cycle because that’s what it really is: bits & bytes —was about a lot of fresh de-novo 0 →1 innovation. A techno-social shift unlocking new human behaviors. Let’s take the example of an early SaaS darling: Dropbox. Before Dropbox, we used to move files between two PCs via USB drives (or emailing it to ourselves). Dropbox was an instant 10x improvement. Now, fast forward to today, your dropbox is no different than my Box, or Office 365 or Google Drive. These products compete on minor differences around branding, support, discounts, or a specific feature or two. That’s an OPEX cycle differentiator, not a CAPEX cycle differentiator.

When we zoom out, this is how much of SaaS looks like today: a Malthusian bloodbath. At the beginning of the SaaS cycle, most markets were green-field opportunities, but today almost every SaaS category is saturated, every account requires a rip & replace, leading to longer and more competitive sales cycles, lower customer loyalties, leading to lower NRR, higher churn, and high vendor fatigue. These are the tell-tale signs of the tail end of an OPEX cycle — a cycle that doesn’t speak “I win because my innovation is better than yours”, but rather “I win because my $s are bigger than yours”.
The first golden age of SaaS is decidedly over.
It’s all about the last inch now and the fight has moved from the high ground to the deep trenches, getting more and more brutal. So, what’s next? If you are building software, what should you be gearing up for?
Enter — the next “mega resource”
For the last 60+ years we have been working our way from manual and industrial automation to compute-driven automation. However, for most practical, multivariate problems, traditional compute alone is highly inefficient. Let me elaborate with a few images below.

Say someone throws you a ball. What do you do to catch it? Take a moment and think: do you really know what you do to catch it? What if you were an android? For a machine, catching a ball in air would be akin to solving complex differential equations followed by a hundred other mechanical complexities pertinent to motion sensors, friction adjustments, actuators and the likes. It would be a massive “compute” problem. How does a human solve it? We don’t solve it via “compute”. We solve it via “insight”, “intelligence”, “instinct”. The ball in the air is asking a question — where will I fall? — and the body, a fine-tuned generalized AI model of hand-leg-eye-brain coordination orchestrated by tiny electrical pulses that have undergone millions of years of training coded into our DNA, answers. We are nature’s original GPTs.
So how does this all tie back to SaaS? All of SaaS today is run on “compute” — a series of algorithms that are then crunched on CPUs and GPUs to reach certain decisions. Take for example a food ordering app. You go on screen after screen to tell the app what you want, another set of screens to enter a checkout flow, then another for tracking the order, perhaps another for calling the agent, maybe one more for support if something goes wrong, and so on. This is how all of SaaS — both B2B and B2C — works today. It’s flowchart and algorithm driven. What if we flip this over its head and go from compute straight to insight? We tell the app “I am hungry” — or heck, not even that! — the app would take just the right inputs from our health apps, our Oura rings, our biomarkers, get our location, time, and day, and know exactly what we may be craving and from which place. Use our CC to place the order (remember, it knows our location!), and even tell the driver to “please leave it at the door right next to that black letter-box!” This, ‘get to the answer’ insight-driven approach is what’s on the horizon. We are already there with search — instead of sifting through dozens of Google links, we get the answer straight from ChatGPT — so why not all of SaaS?

Over the last 60 years, a lot of R&D has gone to bring the cost of compute down to near zero. This led to a personal device in every hand. We also have had the cost of distributing this compute down to near zero as well. That brought the marginal cost of software down to zero, which is basically the reason the internet exists. The next 30 years is going to bring the cost of intelligence down to zero. What would the world of software look like if everything ran on “insights” instead of “compute”? If you are a founder building in SaaS, this is what you should be gearing up for.

Playing the cycles right — a discussion for investors & founders
Just as the earlier Schumpeterian extractive and innovation-heavy cycles unlocked net-new resources — an oil drop, or a CPU cycle — the new resource that is now being unlocked is perhaps an “AI token”. As the internet cycle winds down, and the AI cycle picks up, it’ll be important to know where we are in the cycle and which assets to play in each part of the cycle. As a founder, it likely matters less as a successful founder will likely generate wealth no matter which cycle s/he is in but as a long-term investor, this is important because playing these cycles wrong could lead to investing in the wrong asset or the right asset but at the wrong time. Making these mistakes is more common than we think. Let’s take a few examples. While there has been a 20yr surge in oil production & prices in the US, you’d still lose money in having invested in oil indices over the same period.
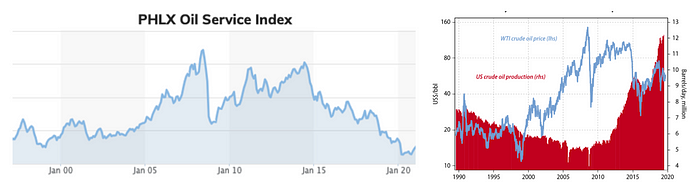
Even in tech, picking the right asset has been important. See below a few market cap growth curves for Amazon, MSFT and IBM. When market cycles are going through its extractive phases, companies that help extract said resources, accrue value. During its distributive phase, those that help distribute said resources accrue value. During its destructive phase, assets get re-priced and value moves elsewhere. For example, in the early days of the internet, a lot of the value was lower in the stack — the protocol and hardware layers — and IBM was king! A majority of its value accrued during this phase because IBM played a pivotal role in making the underlying hardware cheaper, faster, and better. As the protocol and hardware wars settled, the internet entered its distribution-heavy stage: a computer in every home, an internet in every device. The value moved up the stack as the value lower in the stack got more commoditized. Apple, and then Google, Facebook, Amazon took off. IBM simply could not keep up — its market cap post-internet remained somewhat flat over the next 20 years.

Microsoft is a very interesting case study of a company that has played their cards rights during multiple cycles! During the pre-internet phase when world was still in its ‘extractive’ phase around making the underlying protocols and hardware more useful, MSFT owned the operating system and therefore played an important role. During the ‘distributive’ internet era, MSFT owned the browser and therefore maintained its relevance and got a pretty big bump during the internet boom cycle too. After that, a decade+ of more flattish growth till MSFT picked up again the tailwinds of another extractive cycle — first around cloud where it played its card right by making all the right CAPEX investments early during the cloud’s early R&D/extractive phase and then later dominated as SaaS entered its distributive OPEX-heavy cycle which is where we are today. Finally, MSFT is again in the news as we enter yet another extractive cycle around AI.
So what are some of the lessons we can take from these cycles? It is clear that AI is currently in its Schumpeterian ‘extractive’ phase. Companies that help extract this new resource — the ‘Intelligence token’ — are going to accrue more value. What would these companies look like? Just like in the case of Oil, anything that allows the cost of an AI token to come down — so… faster GPUs, better LLMs, better middleware for training or inference, dev-tools, etc, all are going to accrue value (and likely in that order). Nvidia, AMD, OpenAI, Anthropic, Stability, Huggingface, Perplexity, vector databases, LLM security or Ops companies, all fall into this category.
So should everyone just build extraction cycle companies in AI? When is AI going to enter its Ricardian ‘distributive’ phase? Note that these phases don’t happen in a sequence. That said, the reason why many products seem un-investible in AI is because the underlying tech itself is improving so fast that anything you build on top becomes obsolete in a few weeks. This is not a bug, but rather a feature of innovation.
I’ve written about this in more detail here but this goes back to the concept of “slim waists” in technology. Any major technical innovation starts off as a big messy cauldron of ideas. Over years of feedback loops between users and builders, pressure points start to “pinch” at the edges of this cauldron to only allow certain ideas to pass through. Over enough time, these spheres start to look more like hourglasses with the center as the “winning” choice.
Take for example the protocol wars of the early internet — perhaps a dozen of these duked it out before TCP/IP won out leading a Cambrian explosion of hardware and software companies that were built to the TCP/IP spec, or built on top of it. Similarly for microprocessor or OS wars in which x86 (Intel/AMD) and Microsoft/Unix won over the others. Over time large ecosystems were built for/on x86 and MSFT/Unix ecosystems — platform hardware (e.g. Dell, HP, etc), chipsets (Intel, ATI, Broadcom, etc), applications (Google, FB, etc) and more.
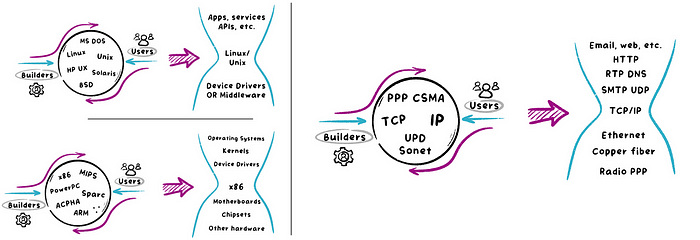
A similar pressure-point needs to first build up for various parts of the generative AI ecosystem across GPUs, LLMs, LLM-infra, devtools, and much more. Right now, there is so much innovation focused on just these layers that these layers are improving almost hourly. Building on top of these layers is like building an airplane when your engine manufacturer is building a better engine every hour. Sure your plane will fly but this is air warfare and your younger competitor already has a faster engine.
The photographs below are from the 1920s (L) & 1950s (R). Just 20 years later, Bernard Sadow “invented” a suitcase on wheels. It only took 5000 years after humans invented wheels and just a year after NASA sent a man to the Moon for someone to think of putting wheels on bags. Sometimes the answers are in front of us but the technology isn’t. Sometimes the technology is, but answers can take generations. All of this goes to say that the opportunity in AI is extremely large but it’ll likely unlock slowly and layer by layer and we are yet to see the truly remarkable use-cases.

For those of you building in the AI space today, I have a few thoughts to offer as a rejoinder.
- My first AI project was back in the early 2000s. I’ve seen a few of these hypes and winters come and go. 2024 will be another winter but AI is a one-way street now. In 2005–10 you could be a social media sceptic and survive as a CMO. No more. In early 2010–2015 you could be a cloud sceptic and survive as a CTO. No more. A few years ago, you could be an AI sceptic and survive as a CEO. No more. You have to be all in.
- If you are building in the ‘extractive’ part of AI, great — $$s will chase you more easily. But you are also likely to suffer from the great ‘pinch-point’ of the hour-glass that’s coming. It’s good to play the picks and shovels game in a gold rush but how many picks and shovels companies do you remember? This is the category that’ll likely see a lot of M&A in the coming years as big platform players will do tuck-ins. As a founder, your job would be to build fast into the available gap today, but then start to sprawl horizontally to build a platform quickly.
- If you are building into the ‘distributive’ part of AI, I guess the obvious solve here is that you need to own some of the data, the models, or own parts of the training or inference stack that gives you an edge over others to begin with. In other words, you need to get closer to the ‘extractive’ part of the layer to get to faster value accretion. Another strategy is to just play the long, long game. Maybe raise less, take a few years to get to scale profitably, and once the lower layers in AI stabilize and we are clearly entering the distributive phase of AI, raise a larger round and scale up!
My guess is that the surprises in AI will reveal themselves when the bottom layers are reaching diminishing returns on innovation and a good deal of the excitement moves further up the layers. When things are moving this fast, I often think of the lone engineer who, unaffected by all the hype, is just looking at the world in a completely new way and as a result, one day, out of boredom, or just sheer luck, genius strikes and years later, as a result, the whole world shifts into a new gear. Good luck to us all in 2024, it’s going to be a wild ride.
Authors