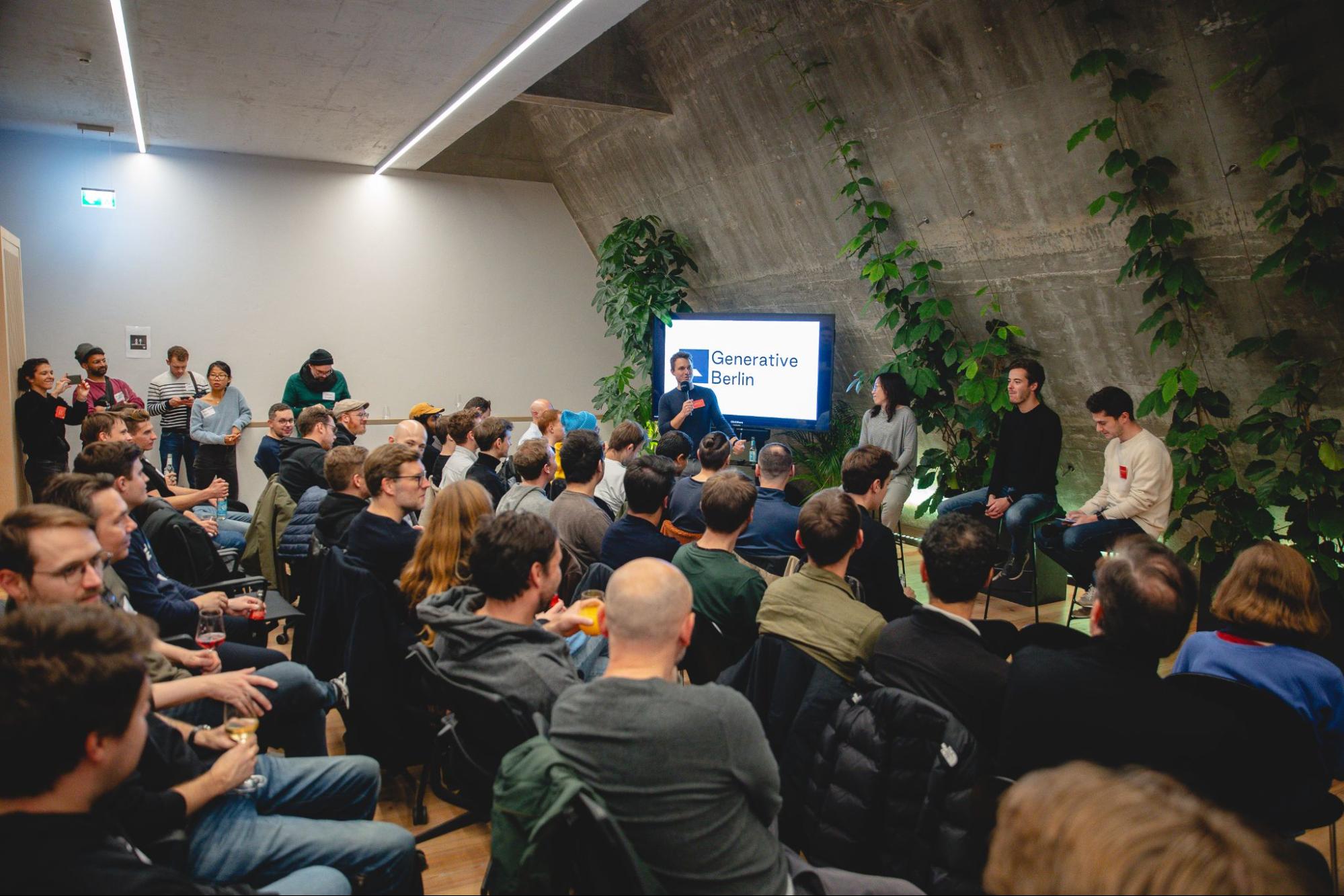
The Generative Europe event series came to Berlin, where Lightspeed took the opportunity to officially open our new office there. At our Paris Generative meetup, we focused on the core infrastructure of building LLMs – so this time we moved up the ladder to discuss the challenges application developers face when bringing LLMs into their applications.
We were fortunate to be joined on stage by Ting Wang, Director of ML & AI at Wayfair, and Marc Klingen, Co-Founder of Langfuse.
Note: If you enjoy going down the rabbit hole like we do, sign up to be notified about our next Generative meetup, here.
Here are four takeaways from our conversation:
1. Experimenting with quick, iterative PoCs with nimble teams
When it comes to experimenting with LLM use cases, taking an exploratory approach is crucial. This is because the field is still in its early stages and there are numerous potential use cases. Ting emphasized the importance of being iterative, explorative, and comfortable with uncertainty.
As a result, the line between a Proof of Concept (PoC) and a Minimum Viable Product (MVP) becomes flexible, and involving early end-user feedback becomes inherent to the early stages of experimentation. This requires from incumbents much more flexibility in how they prototype and design their user experiences. The panelists unanimously agreed that teams experimenting with LLMs need to be interdisciplinary. Given the nascent nature of the field, engineers and scientists must closely collaborate in order to maximize productivity and unlock the full potential of LLMs. In this early stage, rigidly defined scopes can actually hinder progress rather than enhance it.
2. Choosing what to bring into production
The panel highlighted that for productizing LLMs, more traditional approaches can be leveraged. Having a clear understanding of the potential ROI is crucial for making informed decisions, particularly considering the high runtime costs associated with productized LLMs in large organizations. Ting shared her learnings from multiple years at the intersection of data and AI teams. She emphasized the significance of establishing decision-making committees that include not only business and product owners but also scientists. This inclusive approach is necessary to keep up with the rapid pace of development in this field.
The panel discussed that quality control in LLMs ultimately is still an unsolved problem. Consequently, bringing LLMs into production requires a greater willingness to take risks. Especially established enterprises with a high bar for customer quality need to be willing to take sometimes sub-par experience into account.
3. First use cases
Marc, who given his position with Langfuse in the value chain, sees a broad roster of startups as well as enterprises experimenting with LLMs, shared the most common use cases he sees close-to- or in-production. He emphasized that these are mostly use cases that allow for human-in-the-loop feedback with failure-tolerant users. Often they are centered around summarization, recommendation or entity extraction. These use cases have the advantage that model performance can be more objectively evaluated in contrast to more creative tasks where quality is often still perceived subjectively. In contrast, use cases that are directly user-facing are moving to production at a much slower pace due to the high-risk and low oversight. These observations are in line with the recent survey of CIOs from Morgan Stanely (1), where most AI applications are expected to be in production by H2 2024.
Ting shared that good starting points for first use cases are internal processes, where RoI is easier to be defined as well as a closer loop between end-users and development teams can be ensured. Naturally, workflows with high manual involvement but strong repeatability lend themselves as first in line. Concretely these can be use cases in service departments. In e-commerce Ting sees many organizations experimenting with recommendations, extending existing productized traditional ML applications.
The panel highlighted that for wider adoption of end-customer-facing applications, challenges around how to objectize subjective quality evaluation as well as defining a clear RoI and ensuring safety and security remained.
4. The importance of transparency through monitoring
On top of the highlighted stochastic nature of generative models, the panel discussed the importance of creating as much transparency as possible early in the development process. Ting highlighted that as teams are often in the early stages of understanding model behavior and having detailed logging and tracing helps in jointly developing a thesis for use cases. Marc shared how across the application developers he is working with it has become much more important to take an active role in engaging with models. Historically, observability solutions like Datadog have played a passive role in monitoring applications. Hence a new paradigm for application developers emerges: They use monitoring solutions like Langfuse to establish metrics to track across the application lifecycle and continuously monitor while rolling out in production. Observability becomes an enabler to iterate faster for new versions of deployed LLMs. Patterns observed from edge cases in production alter the data sets that the next version of applications is tested on.
We also took this as an opportunity to announce our latest investment into Langfuse. Marc and the team are supporting application developers in this journey of bringing LLMs into production. They are currently hiring their founding engineers.
Authors