02/06/2025
AI
Generative SF: What’s next on the frontiers of crypto, AI, and blockchain
A conversation with Coinbase’s Head of Machine Learning, Rajarshi Gupta, on how AI, crypto, and blockchain are reshaping value exchange, data security, and the future of decentralized tech.
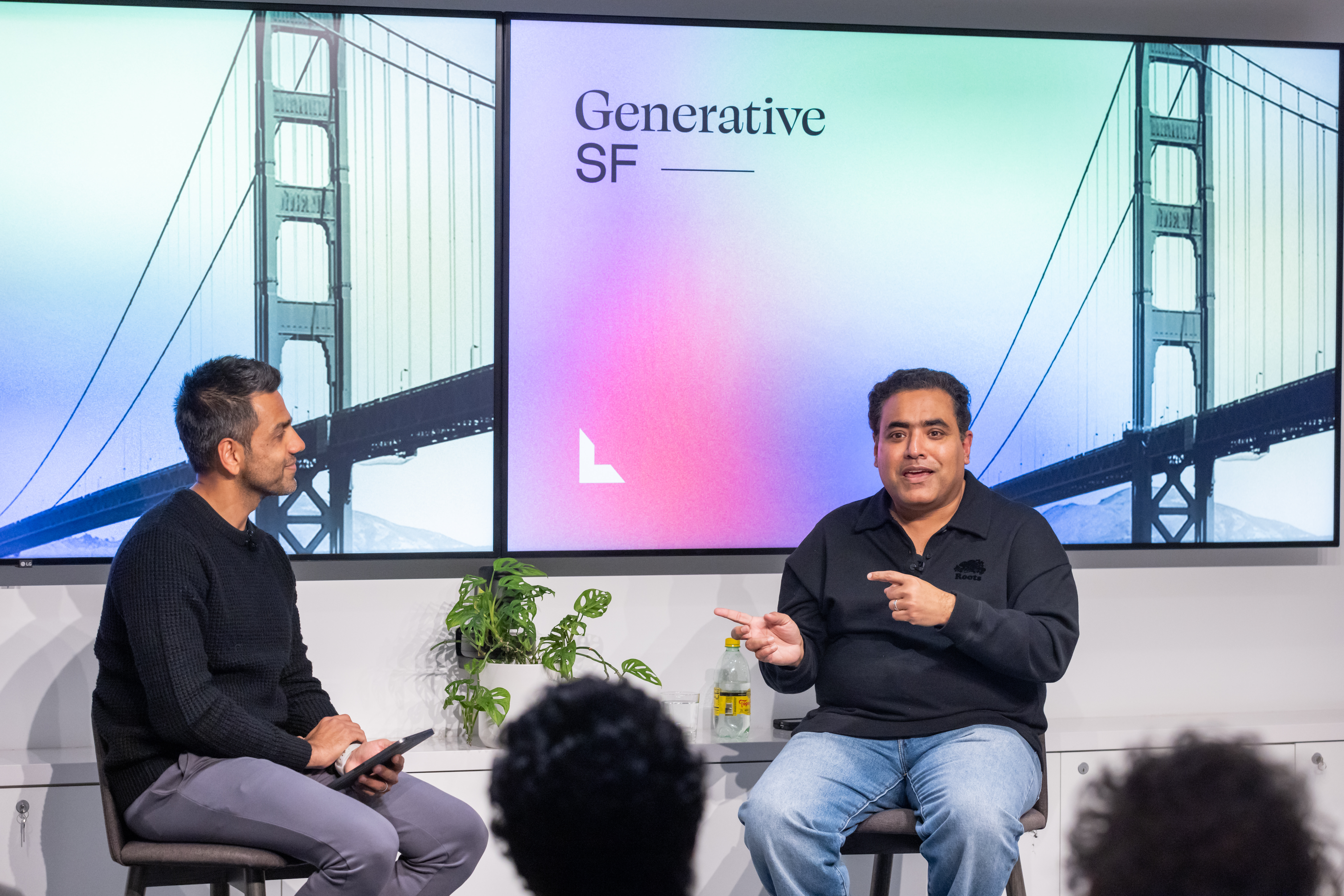
There is no more valuable piece of real estate in tech and business than the intersection of cryptocurrency, AI, and blockchain.
Planted firmly at this critical junction is Coinbase, which has emerged as a pioneering gateway for individuals and institutions venturing into crypto. Rajarshi Gupta, head of machine learning at Coinbase, recently joined Lightspeed Ventures at the last Generative SF meetup of 2024 to talk about how the convergence of these two technologies will impact the future of payments, learning, and blockchain technology.
As a pioneering force with decades of experience in the machine learning and security space, Gupta created the first on-device machine learning engine during his time at Qualcomm Research. Designed to catch Android malware, it shipped on more than 1 billion chips.
In conversation with Lightspeed’s Anand Iyer, Gupta offered a mix of practical experience and big-picture perspectives in areas like enterprise AI, blockchain-based value exchange, secure data storage, and agentic AI. He also discussed the opportunities revealed by CB-GPT, short for Coinbase-GPT, a unified platform for all GenAI applications at Coinbase.
During the hour-long discussion, Gupta reflected on the opportunities and challenges presented through building enterprise-grade GenAI solutions. These challenges included discovering ways to effectively evaluate large language models (LLMs), manage market volatility, and create guardrails that ensure the responsible use of AI. “Internally, we’re facing the same challenges as everyone else,” he said. “It’s a constantly moving space, which can get very hard.”
Here are some takeaways from that conversation.
Enterprise AI: perils and promises
Gupta had a humorous take on the current AI hype: “Every company says they’re doing AI–even the tire shop down the street will claim it’s using AI to change your tires.” However, he noted that successful implementation of enterprise AI is far more elusive.
Enterprise AI initiatives face significant technological and operational hurdles that complicate widespread adoption, including constrained GPU resources. “Getting the available GPUs was our biggest problem last year and caused me the most grief.”
Reimagining value exchange through AI agents
One of the most exciting developments lies in the way AI agents are bridging the gap between AI and blockchain technology. Through powerful new frameworks, AI agents have the ability to facilitate micro-payments and autonomously interact with blockchain networks, opening up new possibilities for automated, crypto-powered applications.
Traditionally, compensation models have been rigid, centralized, and dependent on intermediaries. Blockchain disrupts this by enabling fractionalized, instantaneous payments. AI agents can act autonomously and receive compensation for their contributions in real time. For example, consider an AI agent that performs a complex task, such as data analysis or image generation. Through blockchain, the agent can receive micro-payments immediately upon delivering results, creating a new, decentralized model for blockchain interaction. This evolution challenges long-standing assumptions about labor, compensation, and value creation in the digital economy.
The critical need for secure, decentralized data storage
As AI systems improve, the limitations of traditional cloud storage infrastructure have become more apparent. AI’s immense computational demands and privacy concerns require a new approach. Gupta pointed to decentralized storage solutions as the answer, leveraging blockchain technology to address these issues. “Blockchain is the first technology that makes distributed computing possible because it provides the ability to do it provably, immutably, and anonymously,” Gupta explained.
By distributing data across a network, decentralized storage mitigates risks associated with single points of failure while enhancing privacy protections.
Compliance and regulatory concerns
The regulatory landscape surrounding AI and blockchain is evolving rapidly, so Gupta emphasized the need for organizations to stay ahead of data privacy laws and responsible AI deployment standards. “As an AI leader at a crypto company, I’ve witnessed firsthand the urgent need for such solutions,” he said. Tech companies are under immense pressure to adapt to stringent regulations while maintaining innovation. Organizations face aggressive deadlines and significant consequences for non-compliance, including reputational damage and legal exposure.
To navigate this complex environment, organizations must establish robust governance frameworks and monitoring systems. AI can also play a role in compliance by automating processes, detecting anomalies, and streamlining regulatory reporting. Gupta stressed the importance of creating guardrails to ensure AI systems are deployed responsibly, addressing concerns like bias, privacy, and ethics.
The rise of agentic AI
Agentic AI refers to intelligent systems that can perform complex tasks autonomously, breaking down problems into smaller parts and integrating solutions. This is particularly relevant for industries like blockchain and cryptocurrency.
This emerging age of agentic AI will see the technology move from providing simple answers to working alongside us autonomously, initiating sophisticated interactions that solve complex questions. “This ability to take a problem, break it up into smaller things, and put the answers back together is quite powerful,” Gupta noted. Gartner predicts that by 2028, 33% of enterprise software applications will incorporate agentic AI, up from less than 1% in 2024. This shift could enable up to 15% of daily business decisions autonomously.
Measuring the performance and accuracy of AI systems
ML was built on a foundation of Statistics. “We have grown up with Machine Learning where every prediction comes with its own confidence interval”, said Gupta, “but with LLMs we suddenly don’t know how to measure their accuracy!”
This is an uncomfortable situation, and one that really ought to be solved by deep mathematics. The field of GenAI will progress much faster if we can measure accuracy through robust statistical means. This could be a great challenge for startups, or for academia, and Gupta wanted to encourage the researchers to tackle this intellectually challenging problem.
Implementing AI: strategic tips
Gupta concluded the discussion by offering strategic advice for companies looking to implement AI successfully. He compared AI adoption to learning magic, and pulled in a Harry Potter analogy: “AI really can be like magic, but before you do magic, you have to go to Hogwarts for seven years.”
Successful AI adoption requires companies to invest in infrastructure, data pipelines, as well as hiring skilled AI engineers, training existing teams, and focusing on solving real business problems. “You can’t just put an LLM on a problem, and it’s solved,” he said. “You have to do a lot of work in getting the plumbing right and then doing the testing, measurement, analysis, and iterations.”
Data quality is foundational to effective AI, Gupta said. Companies must clean, organize, and integrate their data sources to ensure models are trained and successfully deployed.
Start with small and focused AI pilot projects, learn from initial deployments, and scale incrementally based on proven outcomes. Collaboration with AI technology providers and startups can accelerate progress, combining internal capabilities with external expertise.
Final thoughts
The intersection of crypto, blockchain, and AI represents a transformative moment in technology. Companies like Coinbase are pushing the boundaries of what is possible, tackling challenges in enterprise AI, decentralized storage, compliance, and agentic systems.
Organizations that embrace this convergence–while maintaining flexibility, responsibility, and innovation–will be best positioned to thrive in this dynamic and rapidly evolving landscape. The future of AI and blockchain isn’t just about technology, Gupta noted. It’s about reimagining the way we work, create value, and interact.
Listen to our latest Generative Now podcast episode to catch this Generative SF discussion.
Interested in attending a Generative event? Sign up here to receive notifications about future meetups, and be sure to listen to the Generative Now podcast, where AI builders talk about how they’re creating the future.