AI is not new in fintech. There is a long history of using artificial intelligence and machine learning in fraud, underwriting, and investing, especially as used by risk teams, lenders, and quant hedge funds.
In fact, the Financial Crimes Enforcement Network (FinCEN) used forms of artificial intelligence back in the early 1990’s to evaluate large volumes cash transactions to identify potential money laundering. This FinCEN Artificial Intelligence System or “FAIS” uncovered over a billion dollars of money laundering within the first few years of operating in 1993.
There is a history, but can also see the present moment clearly: something powerfully new has been unleashed with the latest era of generative AI.
Generative AI is distinct, compared to conventional or predictive AI, in that LLMs can now generate new, creative answers instead of responding solely to specific tasks, do so in a conversational manner, require less supervised training, and require less task-specific training to fine tune the model (“no or few-shot prompting”).
The upside is that generative AI enables chat experiences with AI to feel much more human, almost as if you had another analyst, underwriter, or accountant at your side. At the same time, the advancements in generative AI can pose issues for fintech applications concerned with accuracy.
Fintech is unique in that many use cases have no room for error: finances must deal in precise facts and data. Hallucinating AI is not just a bad user experience, it is a non-starter if its accuracy or math can’t be relied on. The consequences of an incorrect output have real world implications on a person’s identity and financial well being.
Despite the high bar, Lightspeed is long generative AI. Lightspeed has been an investor in AI companies for two decades and we have a portfolio of more than 35 companies with >$850M invested into AI.
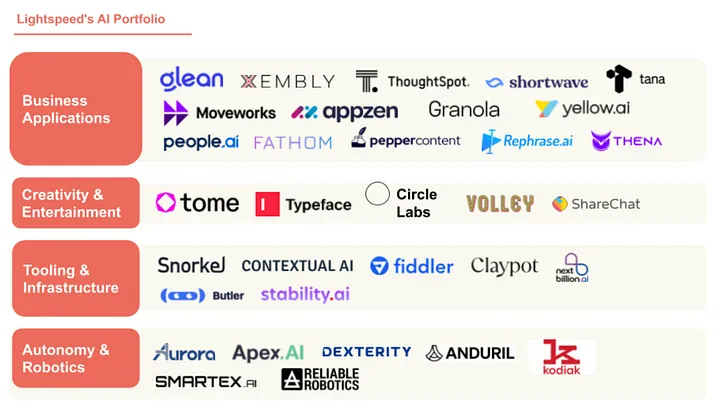
Given the unique attributes and advantages of predictive and generative AI, we asked ourselves: where is each technology best applied? And is there an intersection that enables the best of both worlds?
Predictive AI
Predictive AI excels at identifying patterns and trends in large datasets, enabling accurate forecasting and prediction. It is particularly well-suited for quantitative analysis, risk modeling, and market predictions based on historical data.
Generative AI
Generative AI shines in creative problem-solving and uncovering hidden patterns that may not be evident through traditional predictive models. Its ability to generate new insights and solutions makes it valuable in tackling complex challenges and driving innovation in fintech. From a technical model perspective, generative AI is better than predictive due to few-shot learning (require less task-specific training), text generation, and conversational systems.
We believe combining generative AI and predictive AI will enable companies to reach their fullest potential with AI. By leveraging generative AI’s creativity and predictive AI’s accuracy, financial institutions can maximize their output while maintaining accuracy.
Given this background, we have some specific views about when and where each era of AI can best be used to support fintechs:
- If trained on general data, generative AI best serves as an inspiration starting point. Money is highly emotional. Spending or investing is a high intensity, often personal financial decision. Thus, a tool that more closely mimics speaking with a human will still hold tremendous value in the financial world. Companies building investing research tools and customer service chatbots are great applications.
- If a LLM is trained on domain specific knowledge, generative AI can be used for a broader set of tasks such as document automation, investing research, robo claims, and wealth advisory.
- Predictive AI is likely best used for highly specific tasks like fraud detection, CFO tooling, accounting, compliance, quantitative analysis, risk modeling, and market predictions based on historical data.
- Fintechs can best combine the two by using predictive AI for “back of office” workflows and using generative AI for client-facing “front of office” product experiences. We’re especially interested in finding tools that give users greater control and input over the data and parameters being used to give them a result. The same way consumers have learned to train their Instagram or TikTok algorithm to show them content they want to see or type a specific order of keywords into google to get the most relevant results for them, we believe consumers are already making mental note of what generative AI is best for and changing their questions to elicit the best results. Tools that enable this further will create superior experiences.
We’ve met scores and scores of companies building for this new era. Below is a market map of some of the top areas where we’re seeing applications built and some of our key learnings and observations from our years of collective experience investing in AI companies.
Key Learnings & Observations on building AI for fintech
Frameworks
There are 3 main areas where companies building LLMs and AI interfaces can differentiate:
- data sources (public v. proprietary, domain specific v. general)
- model parameters (training objectives, # parameters, tokenization efficiency)
- user experience (prompt interface / instructions — chain of thought)
Fintech companies can utilize AI to deepen an existing advantage in one of 4 areas: cost to acquire, cost to underwrite, cost to serve, or cost of capital
Where Builders are Creating Today
- The largest segment within fintech (in terms of number of tools built) today is AI-driven research tools (mainly for investing)
- One of the largest revenue generating segment within fintech today is vertical saas fintech applied to a legal use case (personal injury law / insurtech)
- We see large (untapped) use cases in taxes, personal finance and compliance. These are industries with clearly defined parameters that, if fed into an LLM correctly, can deepen a fintechs advantage on all 4 areas mentioned above.
- There is also a large opportunity for a domain-specific security solution for finance LLMs given many enterprises have banned their employees from uploading any proprietary data into
Current limitations to solve
- We’re early in the hype cycle as it relates to AIs impact on fintech.
- Accuracy and data security are the two main concerns fintech buyers share when debating adopting
- Right now companies that are popping up are more geared toward content summarization (e.g. earnings call summaries) vs. value creation (e.g. trading strategies for a value investors compared to swing investors)
- Language models today are still very fragile. A single word out of place can change the output of the model.
- Fintechs will need to invest a lot of money into data science but also back office product infrastructure to support the QA (quality assurance) and data labeling process. In many ways this can become a fintech’s moat, if they have uniquely built out their moderation process.
- Buyers perceive a tension between data security, improving open source tools, and creating the best user experience. Many companies have banned their employees from uploading any company data into chatGPT, even though this would help both the broader network and individual users. Are private, self-developed LLMs the answer?
Building a moat
- Most fintech AI solutions today fall somewhere on the “AI as a feature” to “AI as the core product” continuum. Fewer have material in AI as a core component of the tech build.
- While traditional business moats like brand, UX, distribution, network effects and intellectual property matter, it remains to be seen what moat in fintech AI will prove most useful.
- Data — Because much of today’s financial data is commoditized, fintechs can differentiate with AI by layering in proprietary customer data into the model, amplifying its use case and value. These custom models, tailored with user level financial data, become core to a fintech’s defensibility.
- User acquisition — Because AI systems benefit from more use and more data, the winner in each AI fintech category will likely need to race to acquire as many users as possible. Identifying the right mix of predictive and generative AI will be incredibly important to building a lasting fintech AI company.
What we are looking for in founders building at the intersection of Fintech x AI
When working with founders building in the category, we are looking for teams that put nuance to their thinking of where conventional ML versus generative AI is applicable.
Does your application use case require rigor, precision and is in a zero-mistakes allowed environment? Or are you deploying closer to the end consumer with a more forgiving experience yet the need to offer refreshing thoughts?
While exceptions are always the rule, often fintech founders impress us with a deep understanding of the problem space and bring relevant niche experience. Mixed with a strong technical profile, this allows you to bring a quick go-to-market through nailing the tone as well as providing a technical sound solution.
Lastly, as MOATs are continuously being redefined, teams that are capable of listening, observing and quickly adapting while also being true to their first-principles thinking, have the best chances to succeed.
If you’re a founder building in this space, don’t hesitate to reach out. Join our Generative NYC, SF, and LA meetups to attend an AI builders meeting in person near you. And read our AI reading list. Reach out to the Lightspeed Fintech Team at Fintech_Contact@lsvp.com
— The Lightspeed Fintech Team: Justin Overdorff, Will Kohler, Mercedes Bent, Sam Eisler, Connor Love, Alex Schmitt, and Adrian Radu