09/26/2024
AI
Enterprise
The Future of Voice: Our Thoughts On How It Will Transform Conversational AI
Enterprise Partner Lisa Han delves into why LLMs and multi-modal chatbots are the next leap forward in the evolution of voice.
A little over a decade ago, the movie Her introduced us to Samantha, an AI-powered operating system whose human companion falls in love with her through the sound of her voice.
Back in 2013, this felt like science fiction–maybe even fantasy. But today, it looks more like a product road map. Since ChatGPT launched 18 months ago, we’ve witnessed step-function changes in innovation across a range of modalities, with perhaps voice emerging as a key frontier. And just recently, Open AI launched advanced voice mode, a feature part of ChatGPT that enables near-human-like audio conversations. As such, we are on the brink of a voice revolution, bringing us closer to the Her experience.
Large Language Models (LLMs) and multi-modal chatbots are radically transforming how companies communicate with customers and vice versa. And at Lightspeed, we’ve had dozens of conversations with researchers and founders who are building the next generation of voice applications. Here’s our take on where the voice market is today and where it’s headed in the coming years.
How business found its voice
Commercial voice applications have evolved dramatically over the last 50 years. The first interactive voice response (IVR) system appeared in the 1970s, requiring end users to use a keypad to navigate through voice prompts. In the last two decades, we’ve seen this traditional, touch-tone model give way to something smarter: voice-driven phone trees, allowing customers to use natural language commands instead of just pressing buttons.
Now, we are entering the era of LLM-based systems, where end users don’t just talk to software, but have conversations with it. These systems understand the nuances and context just like a human would.
The Voice AI Opportunity
Today, the IVR market alone is worth $6 billion, and that’s before we consider the broader landscape of voice applications such as audiobooks and podcasts, translation and dubbing, gaming, and companionship apps. We believe the market for voice applications will grow by approximately fourfold, thanks to improvements in latency, tonality, and responsiveness driven by emerging AI architectures.
In the short term, the most successful voice companies will be focused on vertical apps in fields like healthcare and hospitality, as well as apps designed for relatively simple tasks like scheduling. Eventually, however, these new voice apps are poised to wedge their way into broader SaaS platforms, significantly expanding the total addressable market.
Multi-modal chatbots like ChatGPT 4o not only allow organizations to establish closer, more personalized connections with users, they’re also able to collect information around tone, intent, and emotional state, using that data to improve their services and drive new product development.
The current state of voice AI
Currently, AI-driven voice apps rely on three basic reference architectures devoted to ingesting natural language, interpreting it, and generating an intelligent response:
- Speech to Text (STT) ingestion. Capturing spoken words and translating them into text.
- Text-to-Text (TTT) reasoning. Utilizing an LLM to tokenize the text transcription and formulate a written response.
- Text-to-Speech (TTS) generation. Translating that written response into spoken language.
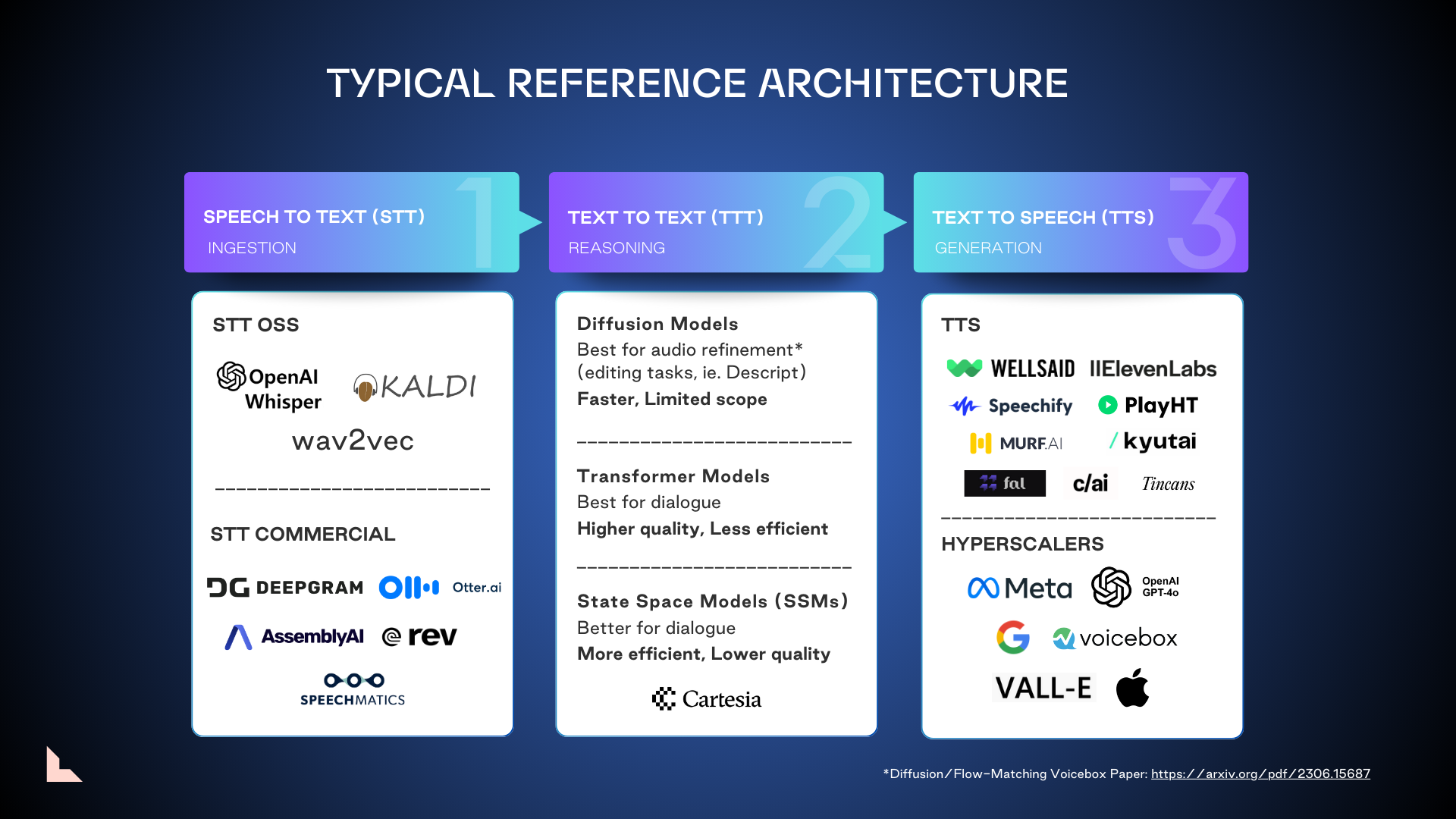
Text-to-text reasoning may involve two categories of models, each with its own strengths, weaknesses, and ideal use cases:
Category 1: Diffusion Models
- Diffusion is an approach to generative modeling and can leverage either Transformers for denoising or SSMs (or both) as the model architecture. They are built by progressively introducing noise to a neural network’s training dataset, then teaching it to reverse this process. While diffusion models can sometimes leverage components of transformers, mainly for interpreting text inputs to condition outputs like images, audio, or video, the generative process itself remains distinctly diffusion-based. These models are faster than other models but more limited; they’re most useful for asynchronous editing and applications like audiobooks and podcasts. Given diffusion models lack reasoning capability, they are often viewed as “garnish” and combined with one or more other models.
Category 2: Autoregressive models like Transformers and State Space Models (SSMs)
- Transformer models work by remembering a sequence of inputs and transforming them into the desired outputs. They are best suited for scenarios requiring nuanced conversational abilities, such as 1:1 dialog or language translation. These models offer higher-quality outputs but require more memory and system resources.
- SSMs respond to the current and most recent prior state of a conversation in time. This makes them faster and cheaper to maintain than memory-intensive transformer models. SSMs also offer lower latency and more lifelike voice reproduction, and they can ingest a much longer sequence of context than Transformers (it’s still to be determined as to whether or not they can really leverage that full sequence in a high-quality way). While they require less memory than transformers, the real challenge lies in leveraging their extended sequence processing for high-quality outputs.
There have been significant strides across TTT, TTS, and S2S. Companies like Cartesia and Kyutai are making significant strides with state space models and open-source solutions. Meanwhile, hyperscalers like Meta Voicebox and OpenAI’s ChatGPT 4o are pushing the envelope with their TTS models.
The next generation of voice AI
When it comes to the next wave of audio AI, there are four top contenders for new audio models, with varying levels of maturity.
1. Latent Acoustic Representation (LAR)
LAR, the model used as the foundation of GPT 4o, enriches acoustic context and delivers higher-quality outputs by not only converting audio into tokenized text, but also picking up metadata like acoustics, tone, and speaker intent. It is easier to train and can be brought to market faster, but not without its limitations.
Because LAR is not trained end-to-end, it’s only suitable for very targeted use cases. Another drawback is that LAR needs to wait for recordings to finish before it can process them, leading to potential latency issues. We consider it a necessary interim step on the path toward tokenized speech models.
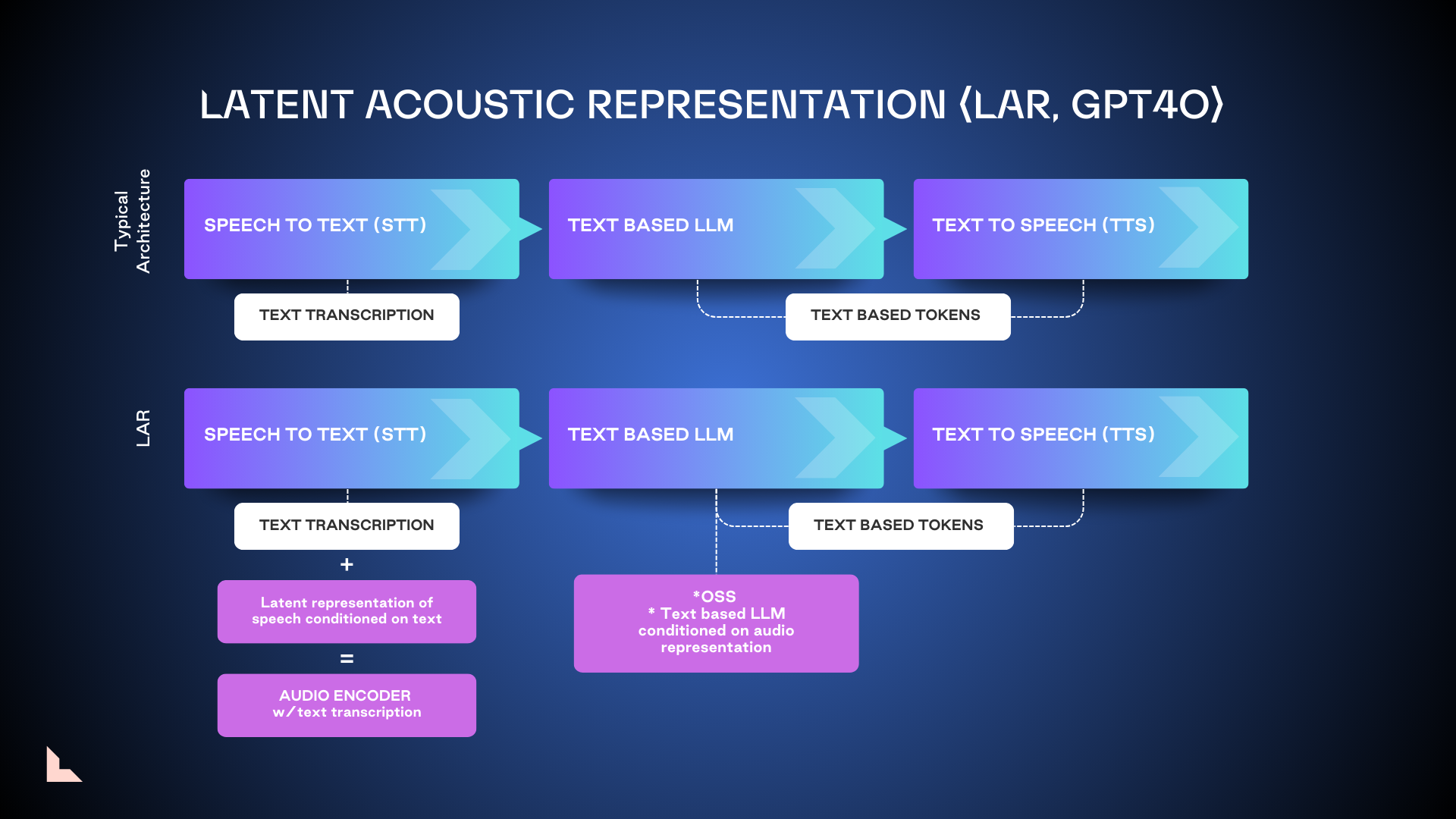
2. Tokenized speech model
Tokenized speech represents a significant leap forward in voice AI technology. These models are already being incorporated into the next iterations of GPT 4.0 and Llama, using both text and audio-based encoders on either end to help yield more accurate outputs. But training these larger models is costly, and there are significant copyright concerns surrounding biometric speech processing. We expect this technology to be market-ready in the next two to three years.
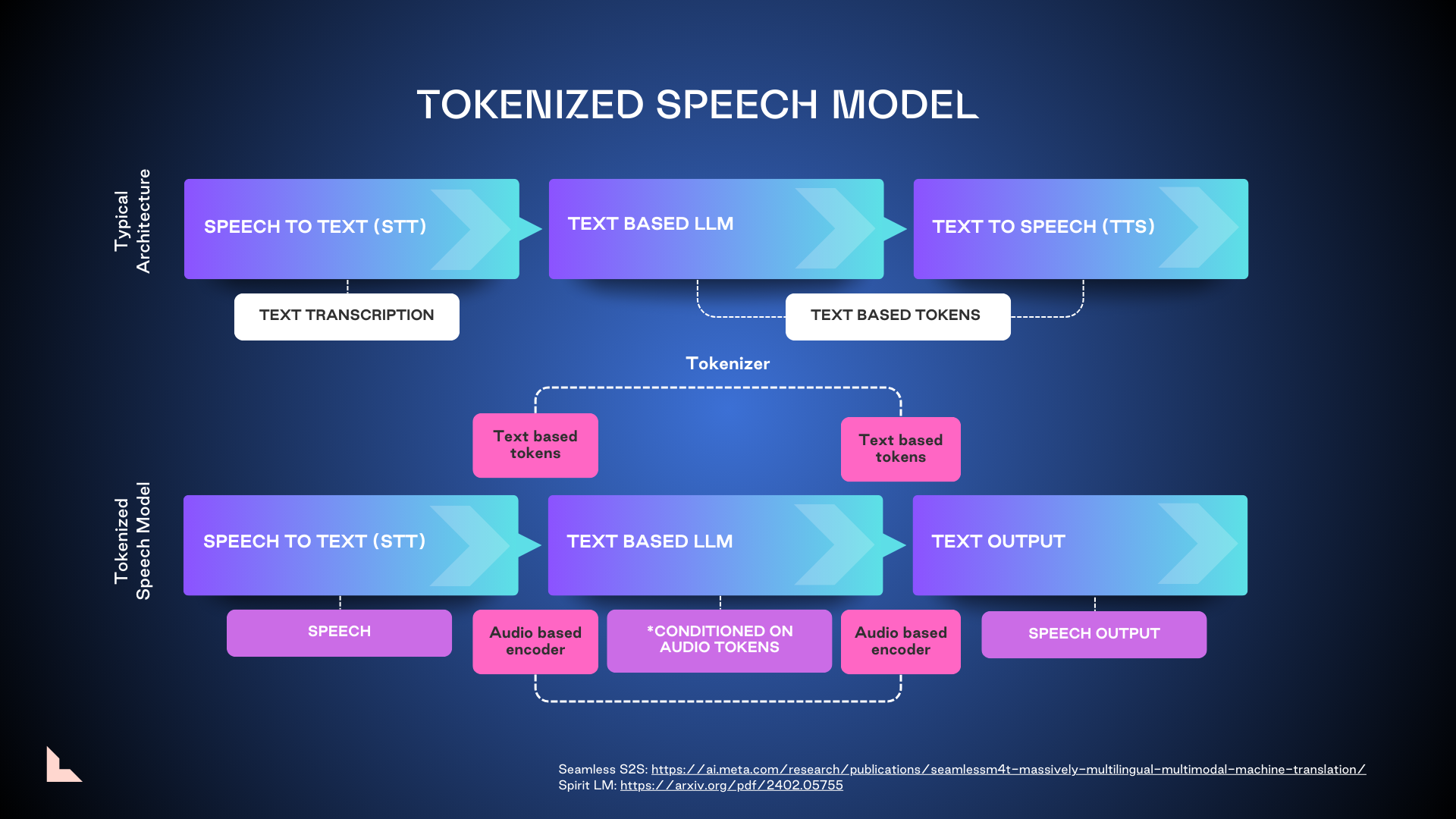
3. Textless speech model
Textless models remove the initial step of transcribing spoken words into text, going directly from the spoken word into an LLM that’s been conditioned on audio tokens. The hope is that removing this step will reduce latency and enable a more human-like experience. However, the research to date indicates that textless models are much more resource-intensive, less performant, and less accurate than tokenized or LAR models.
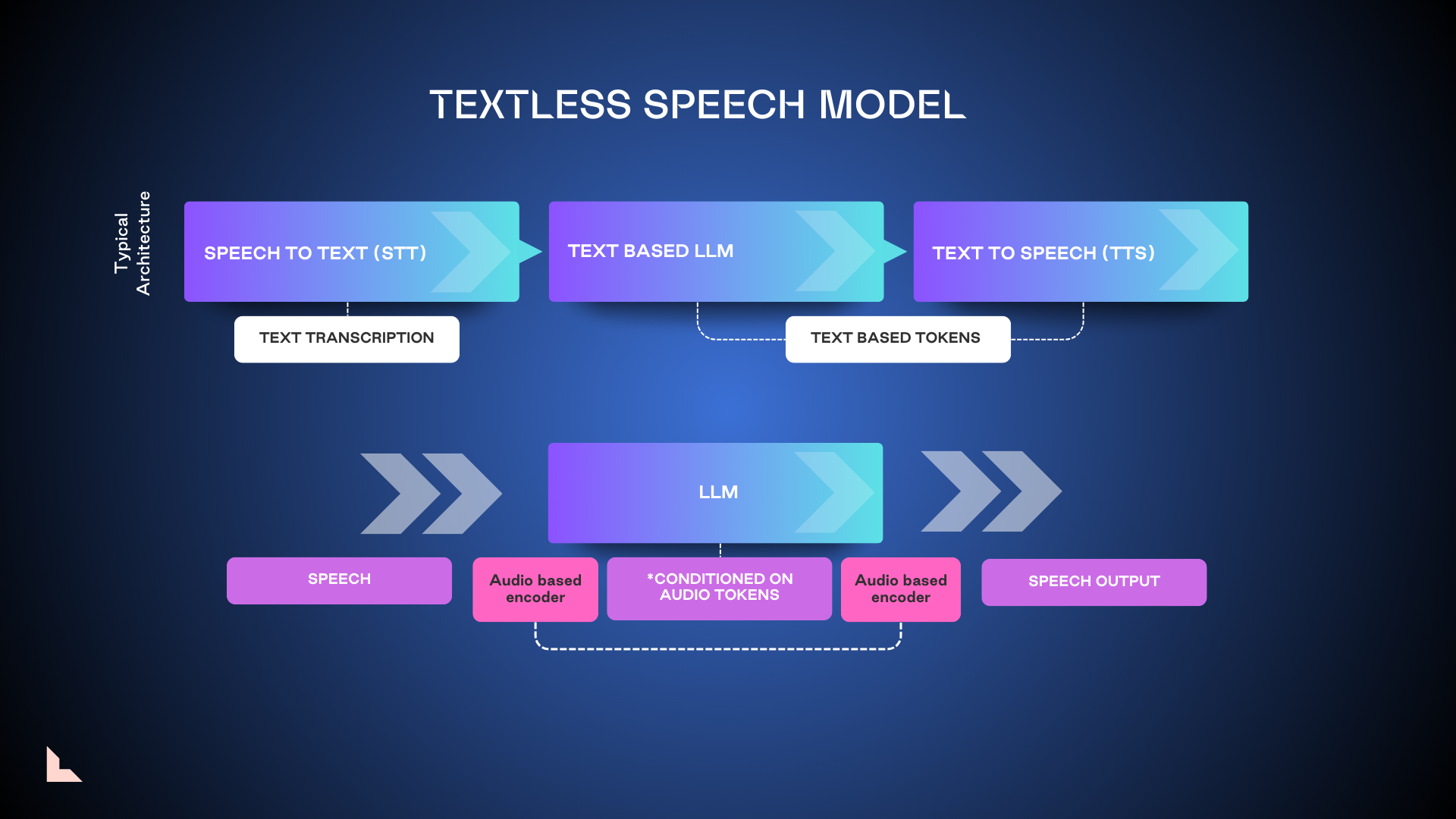
4. Streaming model
Streaming models ingest raw audio streams directly, no tokenization required. This allows developers to configure apps in an always-on state, without the need for conversational turn-taking. While this could potentially speed up voice processing, the always-on nature means it’s likely to be significantly more expensive to operate.
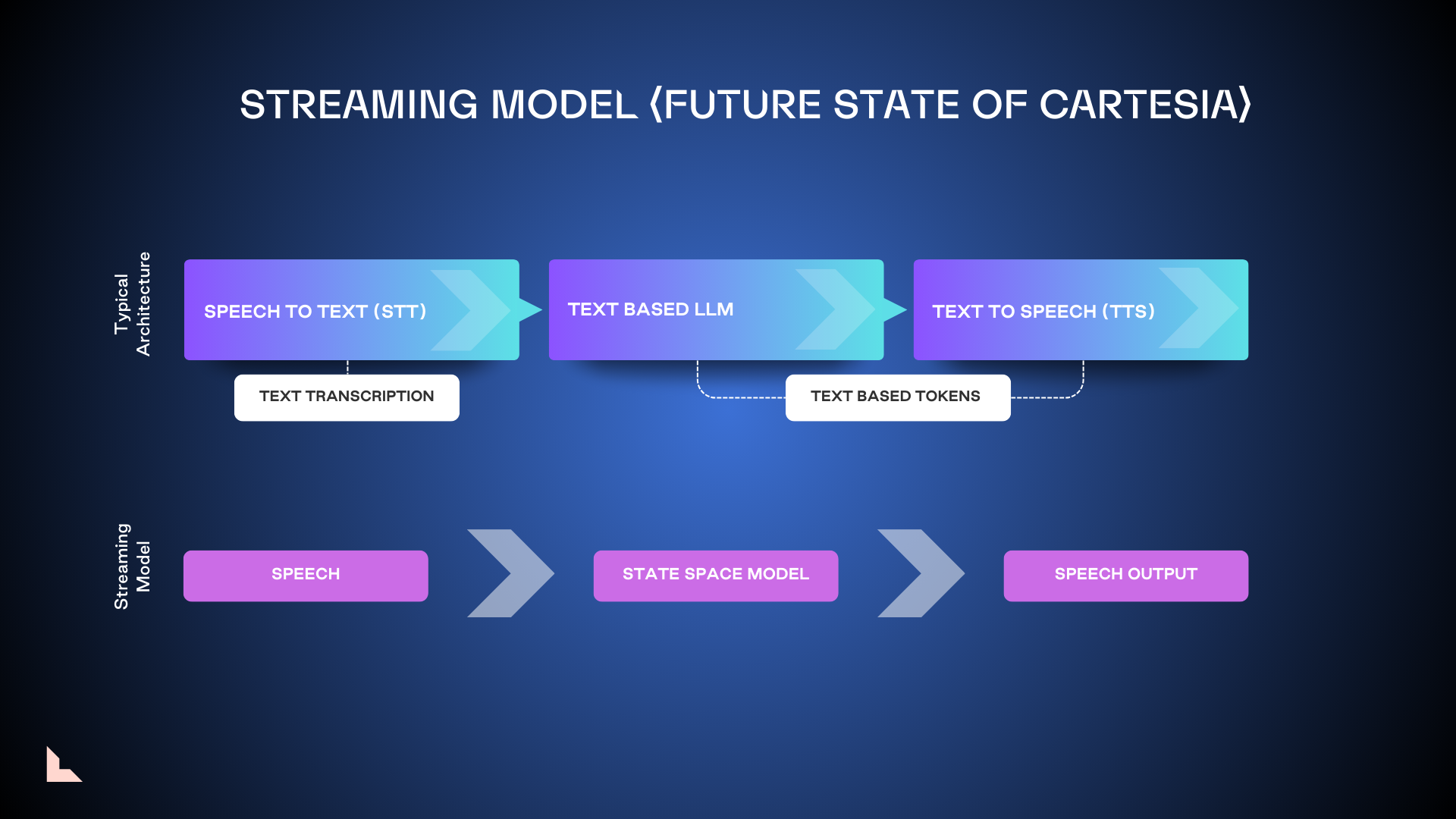
Where the voice AI market is headed and its challenges
Having supported several leading voice AI companies, including Cartesia and Character AI, we believe there are three key challenges voice AI startups will need to overcome before they find their place in the market.
1. LLM first, but with humans in the loop: An important element in any human-AI interaction is recognizing when AI alone is not enough. Applications need the ability to escalate to human personnel seamlessly and efficiently before customers become frustrated. Companies also need the ability to trace root causes when voice apps fail or provide inaccurate information, which requires deep visibility into each layer of the technology.
2. Accelerating performance while lowering latency and cost: Providing near-real-time performance is a challenge for emerging architectures. Tuning generalized models on a per-customer basis at scale is important but can also be time- and resource-intensive. To elevate quality and intelligence, apps using transformer models will need to perform audio tokenization at scale. Reducing latency to sub 250 milliseconds is essential for a realistic conversational experience and is more easily achieved with self-assembling applications rather than full-stack architectures. Cost is always a factor: for voice agents at scale, a difference of a few cents per minute can be significant.
3. Finding the right go-to-market strategy: At this stage, apps aimed at specific vertical markets have an edge over generalized multi-modal AI models. Enterprise-grade applications with strong concentrations in large verticals like healthcare or finance will be able to gain more traction early, though as new architectures emerge and prove their worth, that’s likely to change. A key to early success will be solving the last-mile problem, making the technology easily accessible to consumers and business users.
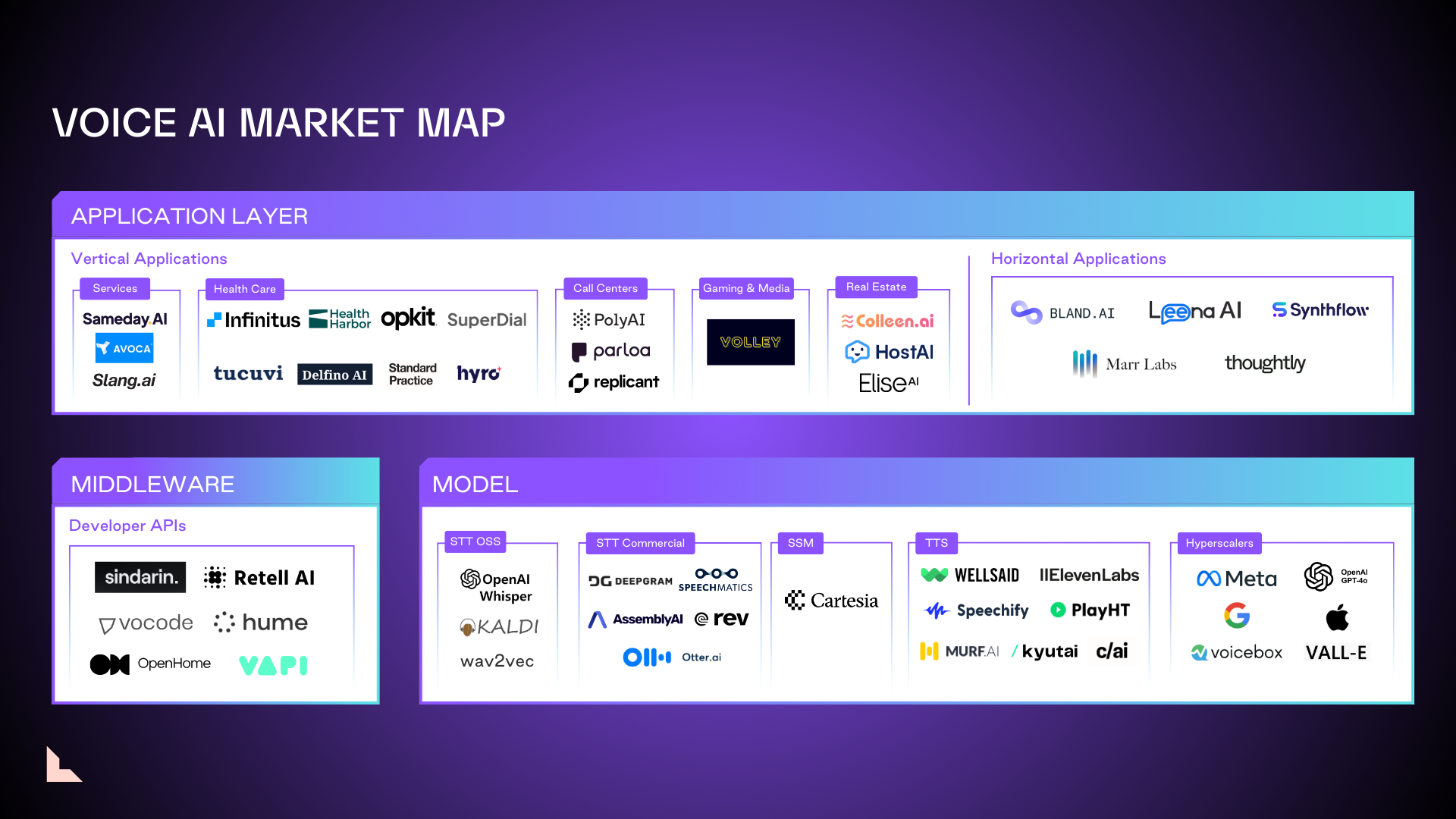
We believe the most effective voice AI approach today uses a model that converts spoken language first into text and then tokenizes it–but one that can also ingest other audio content to deliver a richer, more life-like experience. However, the field is ripe for innovation. There’s still so much to explore, and more research is needed before we can determine if textless and streaming models can overcome their inherent limitations to find a place in the market.
No matter how these technologies develop, we anticipate transformative applications in enterprise audio. People will begin to converse with companies in the same way they interact with their friends today. How organizations deploy voice AI will have an enormous influence on customer satisfaction and brand loyalty, with potentially positive or negative impacts, depending on the chosen tools and execution.
We are not yet at the Her stage of voice technology, where people can develop deeply felt relationships through dialogs with machines, but we’re not that far off from it, either.
JOIN US
Voice technology will revolutionize how we work and play. If you’re a founder with a vision for technologies that can find a place in this exciting and fast-moving market, we’d love to connect. Say hi at lisa@lsvp.com.
Many thanks to Karan Goel (Cartesia), Mati Staniszewski (Eleven Labs), Scott Stephenson (Deepgram), Yossi Adi (Facebook), as well as my colleagues Nnamdi Iregbulem and Raviraj Jain for their valuable contributions to this piece.
Authors